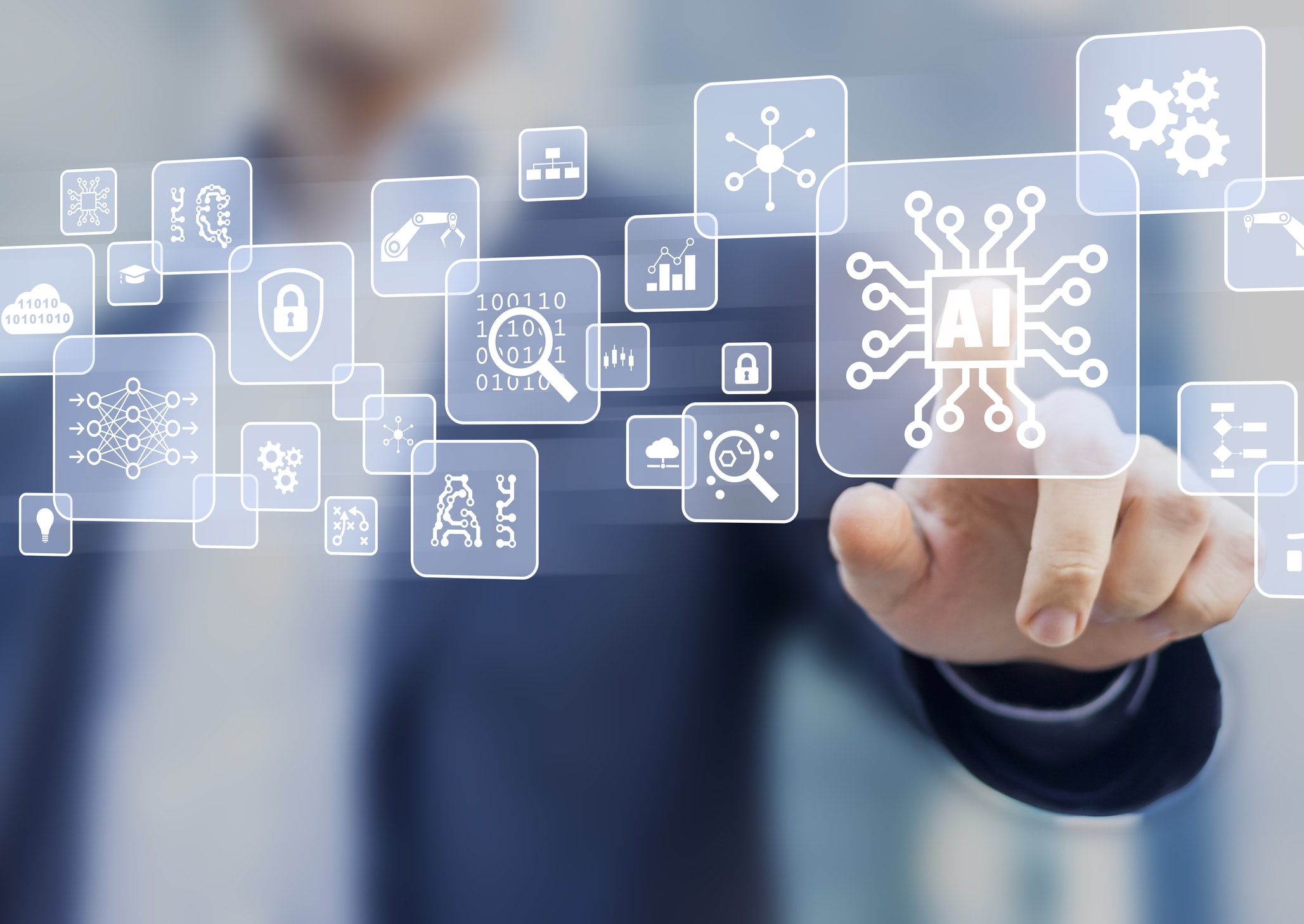
Related Services
DOWNLOAD
DATE
Contact
This article explores the challenge of managing and gaining the most value from big data. We highlight the increased opportunity associated with larger data sets, while illustrating the limitations of current methods and human intellect across the 4 Vs of big data (volume, velocity, variety, and veracity), ultimately resulting in lost value — the fifth V. We further show how organizations can use machine learning (ML) to address these limitations and realize the full value from big data. Finally, we highlight how cutting-edge companies employ ML to obtain greater value.
Challenges in big data applications
Big data is now a commonplace term that represents exponentially growing data. New terms, such as “data exhaust” for all the data being generated by our daily activities and “data lake” for new ways of storing data in its natural format, have also entered our lexicon. In fact, an entire field, data science, has been reinvented and rediscovered just to try to handle this explosive flow of information.
Nevertheless, big data has huge untapped value. The amount of data created in the world is only growing (see Figure 1); our ability to extract value and insight from it should naturally grow with it. Its hidden patterns and clusters will allow us to analyze data sets on a possible cure for tuberculosis or crime prevention, as well as derive more mundane yet valuable insights, such as predicting the value of apartments in urban suburbia or what customers may want to buy and how much they are willing to pay.
However, in recent years the promises of big data have been frustrating. Enterprises wanting to extract hidden value from petabytes of raw data have not managed to do so successfully.The “data-insight-action” chain has suffered from a distinct lack of validation, especially in the context of measurable value.
The reason big data has failed to deliver on these promises in recent years is quite simple: enterprises apply the traditional data analytics techniques they have long used for small data, but now do so with the help of expensive tools with impressivesounding names like Hadoop and Spark. While enterprises can now analyze data at scale and produce impressive and betterlooking interactive dashboards and graphs, they are essentially deriving the same insights as they did a decade ago based on the same type of data and techniques used then. They are effectively unable to consume in a valuable way the plethora of new data available.
Traditional analytics techniques tend to drive analytics functions to be effectively classic reporting functions, regardless of the volume of data or the tools used. Thus, instead of analyzing business intelligence to produce actionable insights, the enterprise produces simplified reports containing sums, counts, averages, and medians, with the occasional SQL query added to the mix.