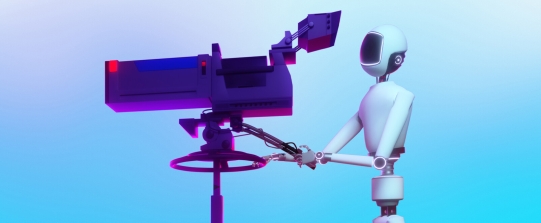
DOWNLOAD
DATE
Contact
The legal and labor-related questions of artificial intelligence (AI) in media and entertainment are still to be decided, but there are many applications that studios can deploy now to boost revenue. For example, postproduction AI applications have the potential to deliver high value to studios without triggering legal or ethical trip wires. Similarly, AI tools can help media producers dramatically reduce risks and lower production costs, increasing the likelihood of market success.
AI is poised to change the world. The full extent of AI’s potential is unknown, but discussions are already high-pitched in the media and entertainment industry, where creative processes have never been easily automated or even quantified. With a fragmented value chain and many manual processes, movie studios and media production companies have yet to unlock the power of data.
The use of AI in the media and entertainment industry is currently a divisive issue. Nevertheless, it has the potential to create a paradigm shift in the industry. This Viewpoint demonstrates how AI can facilitate decision-making and improve processes across each part of the value chain without degrading the value of human creators.
Figure 1 shows the media production value chain (concept to distribution) with the adjacent — yet essential — processes of financing and marketing. Advancements in AI, machine learning (ML), and data science are primed to transform each step of this chain.
Note that data science is a broad, interdisciplinary field that draws from statistics and computer science and uses scientific methods, processes, and algorithms to generate insights from data (see Figure 2). There is overlap with the field of AI, but the terms are not interchangeable. AI involves harnessing computer algorithms to replicate human intelligence and decision-making processes by acquiring, interpreting, and comprehending data.
CONCEPT
Identifying bias in screenplays
Several AI applications have emerged that aim to assist with bias reduction at the concept stage. For example, StoryFit helps production companies simulate how audiences will respond to specific content. StoryFit’s natural language processing (NLP) AI algorithm is trained to pull data from a large number of online sources, reducing the time needed for a query from weeks to hours.
It’s important to note that AI cannot fully eradicate biases, as it is only as unbiased as the data it is trained on and the algorithms it uses for interpretation — AI can only detect biases it has been specifically programmed to recognize. There are also complex legal implications around this type of AI use. (Protections regarding its use were added to the most recent Writers Guild of America’s contract with the Alliance of Motion Picture and Television Producers (AMPTP), and there is continuing litigation from the Authors Guild regarding the use of large language models.)
A more likely application of AI in the concept phase is assisting writers with idea generation. Anecdotal reports of writers using generative AI (GenAI) to create character names and suggest plots and outlines as starting points are already emerging from Hollywood.
CONTENT DEVELOPMENT/SCREENPLAY
Priming content development for success
Data science can play a significant role in this stage of the creative process. Data-driven script analysis can help refine existing scripts and aid in script selection, helping studios greenlight those with the best commercial potential. Since a typical movie script is around 20,000 words, NLP and network text analysis can save studios significant time in early content development.
There are a variety of tools available that predict audience reactions to scripts and recommend script modifications, dramatically streamlining the drafting and editing process. For example, Largo.ai offers suggestions about which type of script edits are needed to achieve the director’s goal, improve commercial viability, and/or widen market appeal. Reports generated by the tool highlight potential revenue risks based on audience/geographic assessment of the script and recommend the best release channels and marketing strategies.
ML can be used to find and fix gender and race biases in films. For example, script text can be analyzed to assess the diversity of a group of characters and whether they are representative of the population in the location in which the film is set. In addition to rapidly tallying character identities, ML tools can assess the number of speaking lines the various groups have, the level of sophistication of the vocabulary they use, and the relative social status of power assigned to the characters by group. These tools are being used to highlight manuscripts with more equitable and diverse representations and/or revise scripts in that direction.
GenAI can create content quickly and efficiently, sometimes with results comparable to human-created content. Recursive learning capabilities have improved AI capabilities to the point where it can be used to reformulate, improve, and expand content, as well as synthesize new material. It can also be used to research topics for thematic content.
AI has the potential to streamline and improve the content-creation process, although there are many ethical and legal questions that still need to be answered, including the role and compensation of the screenwriter and intellectual property rights to the data used to inform the GenAI model. These issues are being discussed, and production studios should be aware of possible copyright infringement and consent liabilities. Nevertheless, given the recent Writers Guild agreement with AMPTP, it’s possible that in coming years, writers may choose to train AI to create scripts in their own style. This would allow GenAI to create an entire script at the writer’s behest using limited prompts, with compensation and credit going to the writer.
PREPRODUCTION
Selecting bankable talent & locations
On every media project, producers and executives are tasked with critical decisions related to casting, locations, and scheduling. Their choices have a significant impact on the project’s cost, schedule, and creative outcome. Traditionally, these decisions rely on human experience (casting directors, location scouts, and production-scheduling experts), but AI can streamline and supplement their decision-making with data-derived insights. AI-based tools can “understand” the context of locations described in scripts and suggest locations where directors can shoot various scenes, saving time spent scouting locations and shooting principal photography.
These tools combine data from media-focused publications, box-office ratings, and industry rewards with filters such as the target age, ethnicity, and gender of the desired audience to generate cast-list suggestions that can be prioritized based on potential revenue generation. Whether these suggestions will be better or comparable to human-generated ideas will be difficult to quantify. Who can definitively say that shooting a film in Georgia was “better” than shooting that film in New York? It’s likely that professionals in this field will leverage the relevant AI tools at this stage of the value chain, rather than be replaced by them. The value unlocked will be time saved in research. It’s also unlikely that decisions without meaningful data behind them — such as casting — will be entrusted to AI.
Knowledge graphs can be a powerful tool for modeling relationships. Knowledge graphs imbue data with intelligence, providing AI with the essential context required for improved explainability, accuracy, and repeatability. Talent agencies are responsible for representing creative professionals and connecting them with opportunities by setting up auditions and negotiating deals, among other tasks. A key challenge faced by many agencies is the centralization of data and knowledge management, with siloed data and limited knowledge sharing.
Knowledge graphs provide a way to merge data silos, creating a comprehensive overview and single source of truth of knowledge in a company, both within and across departments — this is especially valuable for companies with many divisions (e.g., film, television, music, sports, podcasts, social media) across multiple geographies. By leveraging AI and combining both internal knowledge and public data into a knowledge graph, talent agencies can map their clients in a broader context with other talent, media, box office performances, social media perceptions, and traits or genres associated with clients and their work. The context of the relationships between nodes in the graph can create new perspectives for agents in identifying opportunities for their clients, extracting audience and social media insights, talent discovery, or talent/content performance monitoring. These analyses can be used both as tools in negotiating deals and making decisions on behalf of a client, as well as in showcasing powerful analytics capabilities to identify and attract new clients.
PRODUCTION
Spectacular shots at a fraction of the cost
Hollywood studios spend millions hiring experts to operate jibs, cranes, and helicopters to film aerial shots like those seen in popular action movies. More recently, they’ve discovered that AI-enabled drones can autonomously track, follow, and frame the action —delivering smooth shots while flying at precision speeds (or hovering) and deftly avoiding obstacles. When combined with drone management software that allows filmmakers to plan and test routes in advance, these smart devices can drastically reduce production costs.
Taking things a step further, researchers at Carnegie Mellon University, the University of Sao Paulo, and Meta AI asked a few thousand viewers to watch 12 pairs of videos and score them based on how they felt when watching. The resulting data was used to train a model that can direct a drone to mimic the type of shot and movement created by a particular emotion (e.g., fast-moving, tight shots create excitement). As these techniques become more refined, a variety of filmmaking techniques using AI-enabled drones will become possible.
Media producers can also leverage AI-enhanced digital replicas of filming locations to significantly lower costs. For instance, an action movie shot in Dubai can make a digital replica of some or all of the filming locations and have actors complete required reshoots from a studio in the US instead of flying the cast and crew back to the location.
Full-on production replications of actors are another possibility, although this area remains fraught with legal and ethical implications (as demonstrated by the 2023 Screen Actors Guild-American Federation of Television and Radio Artists strike in the US). Nevertheless, with proper consent-honoring rights to publicity and an agreeable compensation package, an AI capture of an actor could save filming days and reshoots on future productions.
POSTPRODUCTION
Revolutionizing international adaptation
Today’s postproduction relies heavily on technology, and AI promises to enhance those processes and methods. A great deal of AI innovation is in sound, with recent breakthroughs in deep learning allowing AI to emulate human voices so well that they breathe, take pauses, and reflect the inconsistency of human voices. This method is not only cost-effective, it’s highly scalable. For automated dialogue replacement (audio recorded in postproduction to fix a spoken line or a remove a background sound picked up during principal photography), actors may well be onboard with using AI-powered tools to cut their time in the studio.
Translation and dubbing is another area with huge potential for AI. When The Champion, a Polish film, was reproduced in English, AI enabled visual alignment of newly recorded English dialogue with the filmed actors’ lip movements. Intelligently dubbed versions of movies and other content in multiple languages (potentially simultaneously) will allow studios to reach previously inaccessible audiences at a reasonable cost.
AI tools can automate other time-consuming postproduction workflows, including video editing. For example, they can help postproduction editors quickly detect an object, color, or emotion in recorded video using automated parameter adjustment. AI can also understand the style and intent of videos and suggest (or create) a suitable background or transition.
DISTRIBUTION
AI-driven matchmaking for distribution success
Data science and graph theory can be used to analyze the factors contributing to successful distribution of a particular movie or show and translate them into effective business decisions. For example, a studio’s distribution strategy often involves deciding whether a title should have a theatrical release or go straight to streaming. Analysis of historical data such as performance of similar titles, along with social listening or sentiment analysis of current customer preferences and perceptions, can provide vital insights into the best channel and windowing strategy.
Knowledge graph modeling of relationships between movie/show elements such as talent, genre, and target audience can help studios identify synergies and opportunities. By identifying similarities in customer base, topic, or talent, these tools make distribution-partner selection faster and more accurate. AI can also help studios optimize release timing by considering variables such as seasonality demand, competitor decisions, location, and demographic factors. Historical and real-time data can be used to model distribution scenarios and determine ways to maximize profits.
Studios are increasingly exploring the benefits of tech-enabled distribution strategies. Warner Bros. signed a deal with Cinelytic to test its platform, which predicts the profitability of a new film by applying AI to a data set of 100,000 movies (cataloged with data related to content, production, and distribution) and 550,000 talent entities (e.g., artists, directors, writers, producers). Similarly, 20th Century Studios partnered with Google to develop an AI tool called “Merlin” that analyzes video frames from movie trailers to match their content to a specific audience by comparing it to a large existing data set.
Of course, success in movie or TV show distribution is not just about analyzing data and applying scientific methods. It requires a deep understanding of the film industry, target audience, and the culture of the country where it will be shown. A successful movie-distribution strategy combines this knowledge with data-driven insights, helping studios identify ideal timing and channels for post-cinematic distribution.
FINANCING
Data-driven insights into trends & opportunities
Developing a budget to secure financing for a movie or TV project is a grueling task, requiring a line producer or production accountant to go through the screenplay line by line, identifying the type of scene, props required, visual and audio effects, and projected costs for directors, producers, and actors.
AI and ML have emerged as valuable tools in this process, enabling fast analysis and accurate prediction of a movie’s potential success. Various data sources such as past box office performance, audience demographics, and critical reviews can be comprehensively analyzed to determine how financially successful a project will be. This type of analysis gives financiers the information they need to make informed investment decisions.
Data science, along with AI, can also play a pivotal role in identifying trends in the film industry, which can greatly influence financing decisions. By examining data on the popularity of specific genres, the performance of movies with similar budgets, and/or the success of movies with similar themes, investors can more easily identify financially viable projects. Data science techniques also help optimize marketing strategies by targeting specific audience segments, increasing the likelihood of a project’s financial success.
MARKETING
Harnessing AI-driven marketing
Compared to other aspects of the media-production value chain, marketing is ahead of the curve when it comes to data use. Decisions made here can make or break a project, and the more data available on the target audience and how its members make their consumption decisions, the better.
Some streaming companies have leveraged their interactive features to gather data on consumer preferences. Netflix’s choose-your-own-adventure Black Mirror: Bandersnatch is a prime example: using A/B click testing, this feature helped the company gather data and analyze decisions about narrative choices, soundtrack, featured products, and more. The engagement data that Netflix stored, alongside other user demographic and decision-making information, can be used to inform audience segmentation and product placement (creating longer-term opportunities for partnerships).
AI can also be used to improve the targeting and effectiveness of film-marketing campaigns. For example, AI can analyze data on past audience demographics and behavior to identify patterns and predict which individuals are most likely to be interested in a specific movie. This can help movie marketers create targeted, highly effective marketing campaigns that reach the right audiences. This technology was successfully used to promote the 2018 blockbuster horror film A Quiet Place, using a platform called Movio that provides demographic and movie-consumption analytics. The film attracted a broader audience than horror films have been able to historically by leveraging AI to identify potential viewers and target women and people over the age of 50.
Additionally, GenAI can be used to create personalized marketing campaigns for specific audience segments. For example, it can be used to create customized ads and promotions based on individual preferences and interests.
As discussed in the section on postproduction, AI in visual effects has many use cases, including creating new opportunities for hyper-personalized advertising. GenAI also has potential to automate and scale social conversations, supplementing the efforts of social media teams.
In summary, Figure 3 shows AI application potential and risk levels at each step in the media production value chain.
Conclusion
UNLOCKING THE POWER OF DATA
Media companies need to evolve their technology capabilities to turn passive, cookie-cutter models into data-intelligent, transformative, value-added ones. They can do this by adopting technologies that leverage AI and ML to provide actionable insights at every point in the value chain. By melding creative raw intelligence with the power of data, agencies and production houses can make cost-effective content without sacrificing creative quality, equitable representation, or box office success.
Here are three things to keep in mind during the process of becoming more data intelligent while maintaining creative integrity:
- Develop a clear understanding of your organization’s processes and operations as a way to identify the areas with the most gaps or operational inefficiencies.
- Identify the areas most likely to realize value from implementing AI technologies. Which tools are the most cost-effective? Which are the fastest and easiest to implement?
- Consider your organization’s long-term goals and overall vision for data-driven media production. Piecemeal software implementations and silo strategies can lead to complications when trying to create synergies between teams and tools.