DATE
May 2017
Design and development of retail banking predictive models
Developing retail predictive models to create personalized commercial offers and increase customers’ value
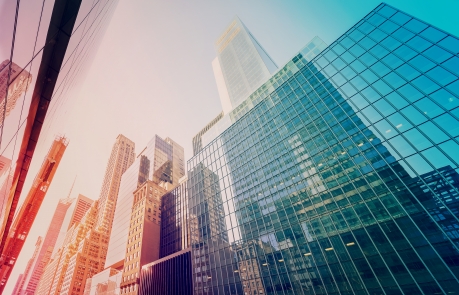
Challenge
The client needed to develop its digitalization strategy to capture new clients, increase service quality and reduce operating costs, in order to strengthen its market position. This could be accomplished through the development of retail predictive models to create personalized commercial offers and increase customers’ value.
Approach
We developed a quick scan of the current database and warehouse in order to validate the data quality, data accessibility and analytical capacities. Then we identified possible predictive models based on channels, client retention and loyalty, and cross-selling; we calculated the impact of each one and counselled the client about feasibility of deployment.
Finally, we selected two models: loyalty predictive model and cross-selling predictive model for mortgage financial products, as well as designing a launching-guide plan to be deployed in the pilot countries, and tracking results.
Value
These predictive models helped the client to clarify its commercial activity over high-value clients and increase cross-selling offers across the client base, identifying suitable clients to attend to with cross-selling offers, probability of client-base churn, proactive levers to retain clients with high probability of churn, and commercial-actions benchmarking in order to boost client engagement.
Design and development of retail banking predictive models
Developing retail predictive models to create personalized commercial offers and increase customers’ value
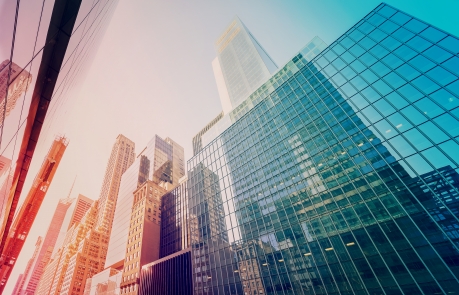
DATE
May 2017
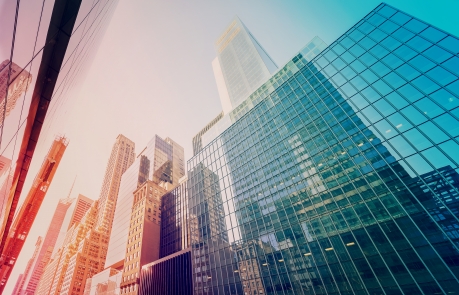
Challenge
The client needed to develop its digitalization strategy to capture new clients, increase service quality and reduce operating costs, in order to strengthen its market position. This could be accomplished through the development of retail predictive models to create personalized commercial offers and increase customers’ value.
Approach
We developed a quick scan of the current database and warehouse in order to validate the data quality, data accessibility and analytical capacities. Then we identified possible predictive models based on channels, client retention and loyalty, and cross-selling; we calculated the impact of each one and counselled the client about feasibility of deployment.
Finally, we selected two models: loyalty predictive model and cross-selling predictive model for mortgage financial products, as well as designing a launching-guide plan to be deployed in the pilot countries, and tracking results.
Value
These predictive models helped the client to clarify its commercial activity over high-value clients and increase cross-selling offers across the client base, identifying suitable clients to attend to with cross-selling offers, probability of client-base churn, proactive levers to retain clients with high probability of churn, and commercial-actions benchmarking in order to boost client engagement.