38 min read •
The Industrial Metaverse
Making the invisible visible to drive sustainable growth
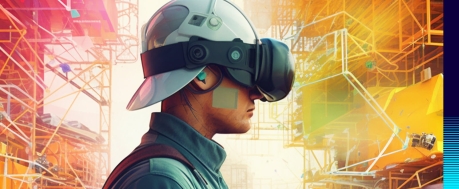
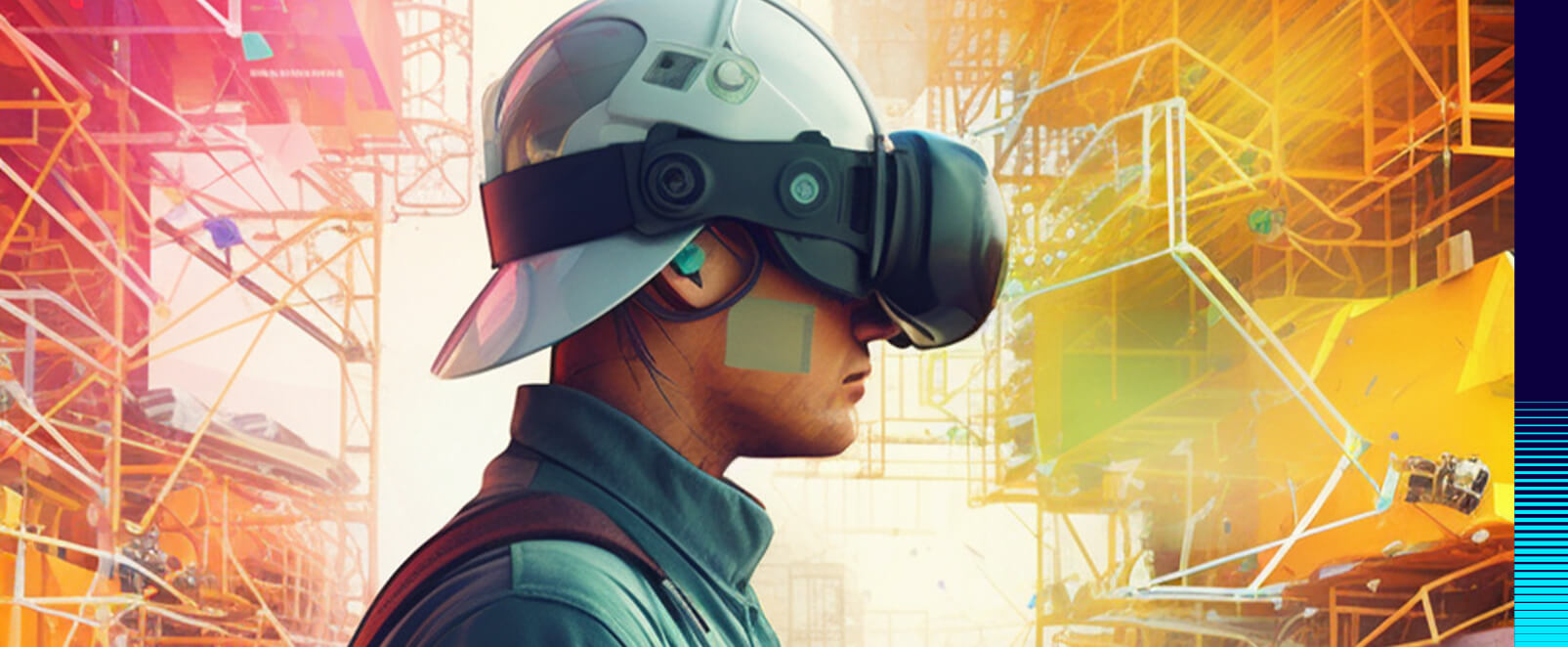
Executive Summary
“What am I? The data? The process that generates it? The relationships between the numbers?”
— Greg Egan, science fiction author, Permutation City
In business and popular media, the Metaverse hype wave is already entering its disillusionment phase, superseded by artificial intelligence (AI). Yet the Industrial Metaverse, perhaps less exciting in the popular imagination, has never really been part of the hype. Is this where the real value of the Metaverse will be realized?
There are differing views about what the Industrial Metaverse is versus the Metaverse as a whole, and how it differs from existing digital twin technologies normally considered under Industry 4.0. In this Report, we provide an evidence-based perspective, assessing current technology status, summarizing use cases and market potential, and offering recommendations for companies going forward.
We conclude that the Industrial Metaverse is best defined as a “connected whole-system digital twin with functionalities to interact with the real system in its environment, allowing decision makers to better understand the past and forecast the future.” As such, the Industrial Metaverse is a further evolution of discrete digital twin technologies that already exist today (e.g., for factories or plants) but progressively extended to ultimately represent an end-to-end, real-world industrial system, including external elements outside the company and the environment within which it operates. The Industrial Metaverse thus provides a transformative tool to elevate the use of digital simulation technology to the level of strategic decision-making. This is important for dealing with the increasing complexity and accelerated pace of development company leaders face and is especially valuable for developing effective sustainable growth strategies.
While achieving a full-scale, connected, end-to-end, whole-system digital twin may be five or more years away — especially due to development gaps in connectivity, computing capacity, and scaled-up AI — intermediate steps are possible in the short term, and many Industrial Metaverse use cases already exist. These can be grouped into four categories: (1) optimization (e.g., digital twins and augmented reality [AR] for operations/maintenance efficiency and productivity improvements); (2) training (e.g., virtual/remote training tools); (3) technical tools (e.g., design/construction/maintenance digital tools); and (4) management tools (e.g., virtual meeting/collaboration/interaction tools). The next development steps will include extending digital simulations beyond discrete physical assets toward multiple connected assets, internal processes, and functions, and finally extended upstream and downstream activities involving the entire industrial system.
We estimate the current Industrial Metaverse market to be around US $100-$150 billion, with a conservative 2030 forecast of around $400 billion but with a potential upside of more than $1 trillion. The benefits to business in terms of productivity could be multiple double-digit percentages. The growth of the Industrial Metaverse will not necessarily depend on widespread adoption of the consumer Metaverse because its utility and value for business depend more on the quality of complex system simulation and less on features such as immersivity and human-machine interface technology. Our conclusion is that the Industrial Metaverse has elements of both evolution and revolution: evolution in terms of the potential for further stepwise penetration of Industry 4.0 technologies, and revolution in terms of how the convergence of these technologies — especially connectivity, AI, complex systems simulation, and visualization powered by increasing computing capacity — has the potential to transform business productivity.
Companies need to consider their strategy for the Industrial Metaverse in the context of their broader digitalization strategy, while also considering implementation barriers. We recommend that companies consider four steps to reap the benefits:
-
Review strategy. Develop a clear picture of the digitalization strategy, journey, and current position.
-
Identify opportunities. Discover value-adding Industrial Metaverse opportunities and develop a roadmap.
-
Implement pilot projects. Adopt a test-and-learn approach and manage change proactively.
-
Build and align the ecosystem. Create a win-win situation with ecosystem partners.
Preamble
When I was a child, 10 or 11 years old, I remember thinking that if it were possible to “scan” the positions and speeds of all the atoms and molecules that make up my body at a given moment and put all this information in a computer capable of simulating all the physico-chemical reactions that govern the universe, then this digital copy would not be distinguishable from the original. There would then be two of “me” — the original, based on carbon chains, and the digital copy, whose substrate would be silicon. The copy would be as conscious as the original, and it would be just as convinced of being me.
I didn’t know it yet, but I had a materialistic approach to consciousness. I didn’t know about Heisenberg’s uncertainty principle, which prohibits knowing with infinite precision the position and the speed of the same particle. Thus, the perfect scan was therefore not possible. Not to mention the computing power required to run such a simulation is still quite far from being available. However, without knowing it, I had conceptualized what the industry would one day call “digital twins.”
Many years later, my friend David Louapre, Scientific Director at Ubisoft and creator of the popular “Science étonnante” YouTube channel, recommended that I read the science fiction book Permutation City by Australian author Greg Egan, released in 1994.
Immersing myself in Permutation City, the digital twin story of my childhood suddenly came back to me like a Proust digital madeleine. Because indeed, one of the key elements of the plot is based on the fact that in the near future, around 2050, it became possible to upload one’s consciousness to a computer. The problem is that in order for a person’s digital twin to be able to interact with a person in the real world, their simulation must run fast enough — that is, enough computing power must be available. If the computing power is insufficient, the time of the simulated person, although remaining subjectively unchanged, passes more slowly than the real time. And if, on the contrary, the computing power is excessive, the simulated world unfolds faster than the real world. It then becomes possible to foresee the future.
These are exactly the objectives that we seek to achieve with the Industrial Metaverse. The Industrial Metaverse is the extension of what has been called “Industry 4.0” for at least a decade. It is the digital twin of a complex system that allows you to project yourself through time and immerse yourself in space. It makes it possible to numerically anticipate the future consequences of a decision or an event on a complex system — whatever this system: a machine, a factory, a company, a value chain.
As we will see in this Report, the Industrial Metaverse has three major advantages over Industry 4.0:
-
Modeling and simulation of complex systems — approaches that were still part of the academic world 10 years ago and that are now changing the game in the industrial world — make it possible to create virtual what-if scenarios. The accessible data is no longer just data from the past and the present, but is now also data about the future. It becomes possible to project in time.
-
Thanks to AI and virtual reality (VR), it finally becomes possible to bring out meaning and visualize the industrial system that must be managed and thus overcome the limits of the human brain, which is not well adapted to apprehend a complex system and its emergences — the famous butterfly effect, resulting from a decision or an event.
-
Interoperability and interconnection between the physical industrial system, its digital twin, and the various stakeholders now, more and more, make it possible to manage it optimally.
Thanks to the Industrial Metaverse, it has become possible to make the invisible visible to drive sustainable growth. While ensuring economic growth, we believe that the Industrial Metaverse will be part of the solution to the climate challenge.
And, actually, it is interesting to note that an anagram of Métavers Industriel (Industrial Metaverse) is:
verdures militantes/militant greenery
This is quite intriguing and, as always, anagrams move in mysterious ways.
– Albert Meige, PhD
1
What is the context for the Industrial Metaverse?
Industrial Metaverse is a term commonly applied to the set of Metaverse applications designed for business users. In our previous Report, “The Metaverse, Beyond Fantasy,” we looked at the Metaverse as a whole, its applications, underlying technologies, and impact. In this Report, we focus specifically on Metaverse applications for businesses and enterprises, therefore excluding applications and experiences for individual consumers (e.g., gaming, entertainment, and social interaction), although there is an overlap where consumers interact with businesses at the customer interface.
Today, the Industrial Metaverse as a concept is both commonly understood and, at the same time, variously interpreted. Business managers are already well-versed in the potential of digitalization, and many are already well along the digital transformation journey. Digital models of physical products and assets, increased connectivity, and new visualizations are very much part of this journey. So what does the Industrial Metaverse really bring in addition? How significant is the creation of an immersive virtual environment to running a typical business? Is Industrial Metaverse really revolutionary, or is it in fact more evolutionary?
In this Report, we examine the background and context of the Industrial Metaverse, define what it means, set out a conceptual architecture, explore its key technological building blocks, assess its value to business both now and in the future, and propose how businesses should go about exploiting its potential. The Report is based on in-house research, client experience, and contributions from interviews with experts across industry and academia.
Industry 4.0 & the Industrial Metaverse today
The Industrial Metaverse is frequently cited as the next phase of evolution after Industry 4.0, moving from cyber-physical systems to a fully virtualized world (see Figure 1).
The term “Industry 4.0” (or the Fourth Industrial Revolution) was popularized around a decade ago and refers to the deployment of a wide range of technologies with the potential to transform industry through new cognitive tools, connectivity, virtual modeling (including digital twins), collaboration tools, and new techniques for manufacturing and supply chain, including advanced robotics and blockchain (see Figure 2).
Of these various technologies, some are especially relevant for the Industrial Metaverse. These include AI, connectivity technologies, virtualization and simulation technologies, and collaboration/interaction tools (see Chapter 3 for further exploration of key technological building blocks).
Industry 4.0 technologies already provide significant benefits to those companies that have successfully deployed them to help transform their businesses. For example, according to data from case examples in Arthur D. Little’s (ADL’s) Operational Excellence Database, these benefits are often double-digit in scale:
-
15%-30% reductions in operational capital deployed
-
10%-30% reductions in supply chain costs
-
30% increased utilization of production capacity
-
10%-40% reductions in maintenance costs
However, overall progress in achieving Industry 4.0 maturity still has a long way to go. For example, a 2020 survey of 70 German companies by Acatech that measured progress against a six-stage Industry 4.0 maturity scale showed that the vast majority of firms (80%) were still in the second stage (connectivity), with only a minority (4%) having progressed toward the next stage of creating digital twins (visibility).[1] No companies had progressed toward the last three maturity stages, which involved modeling complex interactions, simulating future-oriented what-if scenarios, or creating self-governing systems. As we will show later in this Report, these functions are key parts of what the Industrial Metaverse promises to deliver.
It is well-known that making progress on implementation of digital and Industry 4.0 technologies is challenging for any large company. This is because it typically involves fundamental transformation of the way the business operates; it is not possible to simply “bolt on” these new technologies to existing assets, business processes, and ways of working. Typical challenges include:
-
High initial investment, especially in data gathering and management
-
Limitations imposed by legacy IT systems
-
A reluctance to embrace the extent of the required business transformation
-
Difficulties in realizing the targeted business returns from digital investments within short-enough timescales
The Acatech study also highlighted common barriers toward Industry 4.0 progress, including:
-
A lack of common standards
-
Fragile information system integration
-
A reluctance to engage in interdepartmental cooperation
-
Inadequate employee involvement in change processes
If we accept that the Industrial Metaverse is a further stage of evolution beyond Industry 4.0, then it follows that its successful implementation at scale will also require overcoming these common barriers toward Industry 4.0 implementation.
Why the changing business landscape is leading to unmet needs
It is useful to consider how the business landscape has transformed since the early days of Industry 4.0. Today, as well as the constant need to further improve productivity, one of the biggest challenges facing business leaders is how to achieve sustainable net-zero impact growth. Contributing to this challenge are three key factors, as illustrated in Figure 3: complexity, acceleration, and cognition.
Complexity
Industrial systems are increasingly becoming complex systems that are subject to emergent properties making them much harder to manage. A complex system is a system having a large number of:
-
Elements (or parts)
-
Relations (connections between the parts)
-
Nested systems (systems within the system)
Examples of complex systems include cities, the climate, and living organisms. Complex systems differ from complicated systems. Complicated systems run essentially like clockwork, in a predictable manner. They may have many elements, sub-elements, and interactions, but the structure remains stable over time and they lend themselves to problem solving using structured analysis through decomposition of the elements. Up to now, most business management approaches have been based on the idea that a company’s assets, processes, and organization can be approximated to behave, at least in large part, like a complicated system.
However, increasingly this approximation is becoming unrealistic. For example, consider the recent changes in:
-
Elements. In the last two years alone, the volume of enterprise data has risen by over 40% to more than 2 petabytes.[2]
-
Relations. As a proxy for relations, the number of Internet of Things (IoT) connections grew by nearly 20% in 2022 versus 2021, reaching 14.4 billion.[3] Partner ecosystem networks have greatly increased in size and complexity in the last decade. Demonstrating this, the proportion of a typical car manufactured by third-party suppliers increased from 56% in 1985 to about 82% in 2015,[4] a proportion that is still largely the case today.
-
Nested systems. The number of nested “layers” in industrial system architectures has increased. In aerospace, for example, the number of specification elements in the latest passenger jet designs is more than 10x that of its predecessors.
What this means is that the industrial system of any large company — plants, processes, people, finance, customers, supply chain, partners, shareholders, and their environment — increasingly has to be treated as a complex system for management purposes.
Complex systems are inherently difficult to manage due to three specific properties:
-
Emergence. New, unexpected properties emerge from the interactions between the parts.
-
Non-linearity. Feedback loops between the parts may lead to exponential behaviors.
-
Resilience. A small issue within part of the system does not necessarily lead to its failure.
These properties mean that the behaviors of a complex system are very hard to predict, introducing a high degree of uncertainty into the impact of management decisions. Managers relying on simplified models of their systems to help make decisions find that those models are often inadequate. Indeed, failure to adequately recognize inherent uncertainties is one of the main reasons why new IT systems often fail to deliver the expected benefits.
Acceleration
The pace of change for business is continuing to accelerate, causing these unpredictable emergent effects to occur faster and faster. Three factors are driving this acceleration:
-
Knowledge and enabling technologies are being developed and adopted at an increasingly rapid rate, with a greater number of exponential technologies driving transformational change.
-
The lifecycle of companies and products is shortening. For example, the average lifespan of S&P 500 companies has fallen from around 35 years in the 1970s to around 20 years today. Product lifespans in many sectors are reducing, with increasing rates of disruption by new entrants and faster market penetration times.
-
Supply chains are increasingly subject to change and disruption. Ever more complex supply chains and partner ecosystems are being impacted by global and rapid disruptions such as climate change, pandemics, war in Europe, and other geopolitical instabilities. Additionally, sustainability trends such as bio-sourcing are leading to more supplier variability.
This acceleration means that companies need to be able to respond to changing circumstances more rapidly and make strategic decisions faster.
Cognition
The limitations of human cognition mean that making good decisions within these faster-moving, unpredictable systems is difficult. The human brain is not designed to deal well with complex systems — humans tend to think in a Cartesian way, breaking problems into smaller parts, which oversimplifies complexity. In these situations, humans tend to be especially susceptible to cognitive biases — relying on information that matches previous ideas and belief systems — which often leads to incorrect decisions being made.
More and more business phenomena feature nonlinear or exponential behaviors, which the human brain does not evaluate well. For example, a small error in a predicted technology development “curve fit” can cause a big discrepancy down the line, as shown in Figure 4. Examples of this include maturity in autonomous vehicles and nuclear fusion, both of which have been repeatedly overestimated, while recently AI has become exponential after many decades in the flat part of the curve.
Sustainability
Meanwhile, sustainability imperatives mean that end-to-end complex industrial system control is growing in importance. Most developed countries have set goals to be net zero between 2040–2060. This means that companies face the challenge of continuing to achieve economic growth while reducing their environmental impact to net zero.
Achieving progress on net zero impact while maintaining growth requires companies to exert control across the end-to-end complex industrial system (e.g., managing Scope 3 as well as Scope 1 and 2 emissions). This means:
-
Sharing current relevant data (e.g., operational and environmental performance data) across the entire industrial system of which they are a part, including all third parties involved.
-
Being able to predict the sustainability impacts of changes to any part of this system, including supply chain, manufacturing, distribution, sales, in-service, and end-of-life disposal/recycling.
Today this control typically is attempted by conducting discrete impact analyses and collaborating with third parties to share information and take collective action. However, achieving true end-to-end control is difficult due to a mix of technical challenges and institutional, organizational, and cultural barriers around the necessary degree of data sharing and collaboration. On the technical side, the technologies required for gathering, monitoring, analyzing, and simulating the large amounts of data necessary to predict end-to-end sustainability impacts in a complex, large-scale industrial system are not fully mature.
While none of these challenges are completely new to business, approaches used to tackle them today will increasingly be inadequate to meet the needs of the coming years, given current trends.
How the Industrial Metaverse addresses these unmet needs
Industry 4.0 technologies, including digital twins, are already providing significant benefits, but as we have discussed, without further evolution they cannot meet all of tomorrow’s needs for a variety of reasons:
-
They are mainly limited to discrete physical systems, not the “whole system.”
-
Decision-making is too static and siloed.
-
Net zero obligations on industry mean that more effective, whole-system management will be increasingly essential in the coming years.
The Industrial Metaverse provides a way to move beyond Industry 4.0 and overcome challenges around complexity, acceleration, and cognition to deliver sustainable growth by:
-
Allowing companies to make informed C-level decisions based on a dynamic, forward-looking, whole-system approach.
-
Identifying performance improvements and the impacts of change more rapidly and effectively.
-
Helping deliver on obligations to manage overall impacts and achieve sustainable growth.
In the next chapter, we will consider in more depth what Industrial Metaverse means and look at its key components.
2
What does Industrial Metaverse really mean?
In this chapter, our contention is that the concept of the Industrial Metaverse extends far beyond being merely a digital replica of a piece of machinery or a manufacturing plant. It fundamentally serves as a digital reflection of an entire corporation in its operational environment, furnishing decision-makers with insights into historical events and facilitating future predictions. The quintessential role of the Industrial Metaverse is to illuminate the unseen — make the invisible visible. It plays a crucial role in promoting a holistic perspective across compartmentalized units, enabling detailed what-if simulations that provide foresight into potential future scenarios. It unveils the intricate interrelationships within the system. Additionally, it enhances our understanding of the overall behavior and impacts of the entire system, an asset of immense importance for fostering sustainability.
Definition & framework
There are many definitions of what the term “Industrial Metaverse” means, as can be seen in the descriptions shown in Figure 5 from some of the key players contributing to its development.
While there are differing emphases, one element of broad consensus is that Industrial Metaverse is centered on the creation of digitalized models, simulations, or twins of the real world.
Another way to build a useful definition is to consider the technological building blocks that are relevant for the Industrial Metaverse, which, as we have seen, all exist already under the Industry 4.0 umbrella (see Figure 6).
As shown in Figure 6, what makes the Industrial Metaverse distinct from Industry 4.0 is the ongoing development and convergence of many of these technologies. Digital twins, with some form of real data exchange with their physical counterparts and application of AI to assist interpretation and analysis, already exist today (e.g., BMW’s iFACTORY; see also Chapter 4 and Appendix 3). It is the further development and convergence of these technologies — alongside complex system modeling and simulation, data visualization, and collaboration technologies, all enabled by greater computing power — that are leading to the Industrial Metaverse as a new concept able to meet the challenges of complexity, acceleration, and cognition as well as sustainable growth, as set out in Chapter 1.
Based on all of this, we propose the following definition for what, ultimately, the Industrial Metaverse should become:
“The Industrial Metaverse is defined as a connected whole-system digital twin with functionalities to interact with the real system in its environment, allowing decision makers to better understand the past and forecast the future.”
As defined, the Industrial Metaverse has the potential to transform how business decision makers analyze past activities and predict the future at strategic as well as operational levels. We have defined a conceptual framework for the Industrial Metaverse illustrating its key components (see Figure 7).
As shown in the figure, the core of the Industrial Metaverse is the creation and operation of a whole-system digital twin for a real-world industrial system, including all its elements, relations, and layers. Let’s consider each of the key components in turn.
Whole-system digital twin
A whole-system digital twin differs significantly from what we would call a digital twin today, which tends to focus mainly on one or more physical assets, typically plants and products. Its functionalities are representative of an end-to-end real-world industrial system, including external elements outside the company and the broader environment within which it operates. It does not simply cover a factory, plant, or product line and is persistently and bidirectionally connected to the real-world system.
It therefore needs to be constructed as a complex system to enable realistic and dynamic system behaviors to be modeled across all functions, departments, processes, assets, and players. Extensive what-if simulation functionality is a key part of the twin. Simulations are not based on past data alone, but present and future data as well.
While it may include immersive and realistic rendering of a real system, asset, and/or product, this is not the twin’s key defining feature. Visualizations may be in a variety of different forms, depending on the needs for management decision-making.
Real-world industrial system
“Real-world industrial system” refers to the set of elements, relations, and nested systems that represents a business within its environment. It comprises all the strategy, processes, organization, and resources of a business. It stretches across all business functions and assets, including external players such as supply chain and other ecosystem partners, as well as the broader external environment in which the business operates.
By its nature, a real-world industrial system is therefore a complex system, including multiple elements, relations, nested systems, and subsystems, as shown in Figure 8. It is subject to emergent and nonlinear effects.
Connect, compute, conceive & collaborate
The Industrial Metaverse needs to include four key functions to enable a whole-system digital twin that adequately represents the real-world industrial system. These are:
-
Connect — real-time bidirectional connections between the whole-system digital twin and the real-world industrial system to enable data collection and actuation back onto the system.
-
Compute — processing of very large data volumes from the real system, including analytics, complex system modeling, pattern recognition, and simulation, to enable future-scenario formulation.
-
Conceive — visualizations of both physical and nonphysical data, which may or may not be fully immersive. These visualizations interpret and present complex data in different ways, not only to simulate reality but also to facilitate understanding and to illustrate what-if scenarios.
-
Collaborate — functionality to enable a range of interactions, including between internal staff, ecosystem partners, value chain players, customers, and others. Interactions may include commercial transactions as well as everyday coworking.
How the Industrial Metaverse helps meet executive challenges
The essence of the Industrial Metaverse based on the above definition and model is to make the invisible visible. By accomplishing this, Industrial Metaverse offers some key capabilities that are very valuable to C-level decision makers (see Figure 9). Among these, it helps take a systemic view across the silos. It also helps see into the future through enabling realistic what-if simulations. And it helps to make visible the complex interactions across the system. Finally, it offers better visibility on overall whole-system behaviors and impacts, which is invaluable for achieving sustainability.
Industrial Metaverse vs. Industry 5.0
A legitimate question would be: Why speak about the Industrial Metaverse if it is just the next phase of Industry 4.0? Why not call it Industry 5.0?
The answer can be found in the fact that this next phase of Industry 4.0 shares properties with the Metaverse, as we defined it in “The Metaverse, Beyond Fantasy”:
“The future version of the Internet blending the frontiers between reality and virtuality, at the convergence of immersive spaces, collaboration platforms, social experiences, and the creator economy.”
The Industrial Metaverse shares the three key features of the Metaverse that distinguish it from the Internet — namely, immersion, interaction, and persistence — although there are also some differences of emphasis (see Figure 10).
As shown in the figure, immersion and interaction are key for the Consumer Metaverse. Immersion, in the sense of realistic rendering, is still important but not key for the Industrial and Enterprise Metaverse. For example, it is perfectly possible to interpret, analyze, and visualize complex system data without being immersed in a virtual environment. Interaction and persistence are, however, both key for the Industrial and Enterprise Metaverse — business management relies heavily on interaction, and digital twins need to be “permanent,” just like their physical counterparts.
One obvious key difference is that the Industrial Metaverse will generally comprise discrete areas only accessible to authorized persons, rather than the vision of the Metaverse, which is to create an open environment accessible to all. This lowers one of barriers toward greater Metaverse adoption, namely full interoperability.
INTERLUDE
Make the invisible visible
"My art reflects my life, a set of connected yet separate facets, moving together in the digital space.
With a PhD in computational biology from Harvard University and a master’s degree from the Ecole Nor-male Supérieure (ENS), my research centered on computational methods to understand developmental biology. In parallel, I cofounded the nonprofit organization Just One Giant Lab to promote open science across the globe.
Utilizing my expertise in data science and machine learning (ML), I developed musical and visual artistic practices that center around art-science and the question: what is data? A child of the cyberspace, I brought my fascination for immersivity into my research and my art by working with virtual reality as a medium to reveal the invisible threads, from cellular trajectories to the link between projected conscious-ness and avatar embodiment."
— Leo Blondel, Cyber Wizard
echo cos(pi) > /dev/null[5]
Objects are not always what they appear to be. When everything around us is data, what happens when we manipulate it to reveal hidden meanings? The secrets behind complexity can sometime be revealed by changing our perspective. Starting from a pyramid, applying transformation can reveal other shapes in their complexity. In the virtual realm, data is meaning, but only through the eyes of one who seeks to look beyond the visible layer.
Light is matter, matter is light
Modern science has opened the doors to digitizing information in unprecedented ways. By recording the photons emitted by molecules, matter becomes light, which then becomes bytes. Entering the cyber realm, we can shine a new light on reality. In the virtual world, the limits are bounded only by the imagination. Here, an embryo simulated from light data becomes matter again, with simulated photons coloring its invisible matter. From a transparent embryo, where light goes through only slightly scattered, the digital process allows the generation of an opaque object, upon which light will reflect: from transparency to opacity, revealing invisible shapes and processes.
3
Where is Industrial Metaverse technology today?
In this chapter, we explore the technological building blocks of the Industrial Metaverse: their maturity, challenges to overcome, and what this means for the timescales at which the full potential of the Industrial Metaverse could be realized by business.
Evolution of technologies relevant for Industrial Metaverse
It is helpful to begin by considering relevant technologies that are already in place in industry as a result of the wave of computerization and connectivity that has been ongoing for at least three decades (see Figure 11).
Technologies that involve digital simulation and virtualization, such as building information management (BIM), computer-assisted design (CAD), and digital process control, are already mature although still not fully deployed. The use of AR (e.g., for improving asset construction, maintenance, and inspection) has also become well-established, although there is still further to go in terms of adoption by industry.
In more recent years, digital twins to represent plants, factories, and products have started to be deployed, although often still more as pilot schemes and trials (see also Chapter 4 and Appendix 3 for selected use cases). These are already being used effectively to improve operational efficiency, maintenance, and quality. And in many industries, virtual simulations are already being used for operational training.
In this sense, therefore, aspects of the Industrial Metaverse already exist, overlapping with Industry 4.0, as shown in Figure 11. However, the availability of “connected whole-system digital twins with functionalities to interact with the real system in its environment,” as per our definition in Chapter 2, is still five or more years away (we provide more detailed analysis later in this chapter).
Moving toward connected whole-system digital twins
There is a fairly clear path of evolution toward whole-system digital twins that involves progressively extending and integrating digital simulation beyond discrete plant operations. Starting with technology that is already deployed today, this could mean, for example, the following steps:
-
Step 1: Digital twins for discrete, selected plant operations — including the use of AI-enabled data analytics, increased automation, AR tools for maintenance, and virtual training
-
Step 2: Digital twins created for multiple plant operations — including supply chain linkages and data integration; increasing the extent of simulation, such as by including sourcing and/or logistics optimization
-
Step 3: Digital twins created for the whole operational system — including upstream and downstream players. In this step, various management processes are increasingly integrated into the simulation in addition to purely operational processes
-
Step 4: Digital twins created for the whole end-to-end industrial system — including all management and operational processes, assets, people, and the environment
Some early examples of Step 2 already exist today. Michelin, for instance, has already piloted an approach that involves digital twin simulation for global sourcing strategy optimization, enabling the company to test and compare different strategies for optimization across a range of parameters and achieving impressive results (see sidebar “Michelin: Evolving digital twins beyond plant level to optimize global sourcing strategy”).
Technology building blocks — Maturity & further development needs
Realizing the next phase of the Industrial Metaverse at a whole-system scale will require further development and integration across a range of technology building blocks, as outlined in Figure 12, and must consider the four functions we discussed earlier:
-
Connect — requires bidirectional data exchange between the digital twin and the real-world system, comprising both “hot” current data via IoT technology and “cold” stored data via enterprise resource planning (ERP) systems.
-
Compute — requires technology to simulate complex systems and enable future scenarios to be run. It also leverages AI for data analytics and interpretation, especially for prediction.
-
Conceive — requires VR, AR, and mixed reality (MR) visualization technologies, as well as other types of visualization for complex data interpretation, sometimes integrated with AI. Advanced human-machine interface (HMI) technologies are also a key building block.
-
Collaborate — requires a range of technologies, including enterprise collaboration tools (such as Mesh and Horizon in use today), and transaction-based tools such as those often linked to Web3, including blockchain and non-fungible tokens (NFTs). API architecture that ensures easy software interoperability and data exchange is also important.
Underpinning all this functionality is the need for increased computing capability, which involves a wide range of developing technologies at different levels in the stack, from new chip design, quantum computing, and high-performance computing through to cloud and edge infrastructures and availability of low-code/no-code software solutions.
Table 1 summarizes our assessment of the maturity levels of each of these technology building blocks, including key development needs and timescales to full maturity.
In Table 1, “scale of development gap” refers to the extent of development still needed to be able to deliver the functionality envisaged for adoption of large-scale whole-system connected digital twins. “Degree of uncertainty” refers to the likelihood that current challenges may not be overcome during development. It may be seen that for the most part, the various technology building blocks have only moderate development gaps. All have only moderate degrees of uncertainty in terms of if/when current challenges will be overcome.
Overall, the picture suggests a timescale of five or more years before whole-system digital twins as described in our Industrial Metaverse definition could be realized, although in the meantime there will be continued progress with intermediate steps. AI is assessed as having a larger development gap than others mainly due to the challenges of algorithm scalability, data volumes, and computing-capacity limitations. Computing capability itself is a critical enabler for achieving very large, connected digital twin simulations. For example, many optimization problems involving multiple elements and interactions qualify as “intractable problems,” where the solution complexity rises exponentially beyond the reach of conventional computing power. Such problems would be suitable for quantum computing approaches, but this technology is probably at least 10 years away from maturity.
In the section that follows, we provide more details of the status, maturity, and implications for the Industrial Metaverse of each of these technology building blocks.
4
What is the potential value of the Industrial Metaverse to business?
In this chapter, we assess the size and future growth of the Industrial Metaverse market, provide an overview of current use cases across different functional categories and industries, and look at potential future use cases that could be possible when the Industrial Metaverse evolves further.
Market size of the Industrial Metaverse
In order to assess the size of the Industrial Metaverse market, it is important to be clear on what is included and excluded.
We have defined the Industrial Metaverse market to include the following:
-
All of the digital twin market
-
The industrial segment of the VR/AR/MR market, excluding the consumer segment
-
Most of the IoT, AI, and blockchain markets (>50%, depending on definitions)
Other Industry 4.0 technologies such as robotics and 3D printing are excluded. Computing and telecoms infrastructure markets are also excluded, as they are relevant for the entire digital market.
Based on this scope, we have looked at a range of market forecasts from different sources to indicate market potential (see Figure 13). Note that we have not conducted any bottom-up primary research.
As shown in Figure 13, the Industrial Metaverse market size in 2023 is in the $100-$150 billion range. This is smaller than many existing Industry 4.0 market estimations, which is logical given that it excludes technologies such as robotics and 3D printing.
There is much variability in forecast market growth from Industry 4.0 from different sources, and, indeed, any such forecasts should be treated with a great deal of caution. Our conservative 2030 forecast for the Industrial Metaverse market is $400 billion, although the upside could be >$1 trillion, with CAGR between 20% and 30%.
This range is wide but in line with previous ADL estimates of the broader Metaverse, which included a conservative $500 billion low end and a multi-trillion-dollar high end (see “The Metaverse, Beyond Fantasy”). Irrespective of the actual number in eight to 10 years’ time, it is clear that the market potential is very significant.
Current Industrial Metaverse use cases
Today’s Industrial Metaverse use cases can be broadly divided into four categories: optimization, training, technical tools, and management tools. Each of these has a number of subcategories, as follows:
Optimization
-
Plant/line operational-efficiency improvements
-
Predictive maintenance optimization
-
Product-quality optimization
-
Supply chain operational improvements
-
Sales operational improvement
-
Customer service improvements
Training
-
Operational staff training
-
Safety and emergency training
-
Remote training
-
Product training
Technical tools
-
Design/construction integration and design tools
-
Asset inspection/maintenance tools
-
Remote asset troubleshooting/problem-solving
-
Advanced data analytics
-
BIM
Management tools
-
Virtual meeting tools
-
Virtual collaboration/workshop tools
-
Customer-interaction tool
These use case categories stretch across most business functions of a typical company, as shown in Figure 14.
Current use cases cover the first stage of maturity of the Industrial Metaverse, overlapping with Industry 4.0. Please see Appendix 3 for a non-exhaustive list of 30 use cases based on real-world examples and organized by industry, category, and aim. These are illustrated in Figure 15.
Future Industrial Metaverse use cases
Taking an in-depth look at future use cases demonstrates that they provide major breakthrough benefits to large companies by enhancing strategic management effectiveness, as shown in Figure 16.
Although it is impossible at this stage to predict absolute numbers, it is safe to assume that they could deliver double-digit percentage performance improvements.
Current players in the Industrial Metaverse
There are hundreds of companies currently active in providing the technologies that underpin the Industrial Metaverse. Figure 17 shows a limited selection of these companies, grouped according to the technology building blocks outlined in Chapter 3. We share more detailed profiles of selected Industrial Metaverse/digital twin players in Appendix 2.
It should be noted that many players are involved in multiple technology building blocks. Obviously, many of these companies are also involved in developing the architecture of the wider Metaverse, as set out in “The Metaverse, Beyond Fantasy.”
Recent market movements in the Industrial Metaverse
The Industrial Metaverse market is still relatively immature, with predicted major growth likely to come in the medium term. Given the current pressures on the technology industry, and the Industrial Metaverse’s current lack of significant revenues, this has led to the scaling back of some initiatives as part of wider technology industry downsizing. For example:
-
In November 2022, Meta announced that it would lay off 11,000 employees, 13% of its workforce. Plans to cut a further 10,000 employees were announced in April 2023.
-
In February 2023, Microsoft shut down its 100-person Industrial Metaverse team, founded just four months earlier, as well as closing its AltspaceVR and Mixed Reality Toolkit teams. This was part of a wider 10,000-person downsizing initiative. However, a Microsoft spokesperson stated the company “remains committed to the Industrial Metaverse.”[15]
At the same time, multiple companies have recently announced partnerships:
-
Microsoft/Meta (2022) — product integration between Teams, Office, and Windows software and Meta’s VR headsets
-
Microsoft/NVIDIA (2022) — collaboration on AI, including cloud AI computing, AI applications, and services
-
Siemens/NVIDIA (2022) — connecting the NVIDIA Omniverse and Siemens Xcelerator platforms to enable full-fidelity digital twins and software-defined AI systems. The partnership is focused on the manufacturing industry
-
Unity/Hyundai (2022) — developing Meta-Factory, a Metaverse-based digital twin factory to optimize plant operation and allow virtual problem solving
From these recent events, it seems reasonable to conclude that, despite short-term setbacks and retrenchments, which reflect the prevailing global economic climate, the longer-term drivers for Industrial Metaverse growth remain strong. As we have shown, the business benefits are significant, and the barriers toward adoption are less dependent on key obstacles for the broader Metaverse, such as consumer willingness to engage in immersivity and level of realism.
5
What should companies do?
In this chapter, we draw some overall conclusions on where the Industrial Metaverse is today and look at what companies should do to ensure that they respond appropriately to ongoing developments and reap the benefits.
Overall conclusions
The extended and enhanced use of digital twins is at the core of the Industrial Metaverse. Digital twin technology has already existed for many years and is part of many digitalization and Industry 4.0 transformation programs. In this sense, therefore, the first stage of the Industrial Metaverse is already well underway. This is a source of confusion in many discussions where people question whether the Industrial Metaverse is really anything new, or if it is instead just hype. It is the extension of digital twin concepts to cover the entire organization at operational, management, and strategic levels that is the essence of what the Industrial Metaverse will bring in the coming years.
The Industrial Metaverse does not depend fully on general Metaverse adoption in order to be successful. Many Industrial Metaverse applications, such as the use of digital twins for operational improvement, are not heavily reliant on a “perfect” rendering of reality or providing a truly immersive user experience. They also do not depend on full interoperability between competing Metaverse worlds. In this sense, further progress is possible even if adoption of the consumer Metaverse fails to take off, as some observers have predicted.
The convergence of several key technologies is providing a strong driver for Industrial Metaverse development in the coming years. The convergence of further technology developments in real data connectivity, AI, data visualization, collaboration, and complex system simulation, powered by new computing capacity, acts as a powerful driver for progress in the Industrial Metaverse. This is despite shorter-term barriers resulting from global economic conditions.
The key technologies for achieving extended whole-system digital twins are not yet mature, and there are other barriers including data sharing between players. While progress is accelerating in areas such as AI, realizing the next stage of development of the Industrial Metaverse will require further development across all key technologies, as well as overcoming barriers that prevent data sharing between players in an industrial system.
Moving ahead with the Industrial Metaverse is inextricably linked to the digitalization journey. Companies therefore need to consider their strategy for the Industrial Metaverse in the context of their broader digitalization strategy. It will usually not be possible to “leapfrog” to Industrial Metaverse implementation unless digitalization is already fairly mature.
How companies should respond
Essentially, the approach companies should take for moving forward on the Industrial Metaverse should be based around normal IT or ERP system implementation good practice. Generically, we suggest considering four main steps (see Figure 18).
Review strategy: Develop a clear picture of the digitalization strategy, journey & current position
As a starting point, companies must have a clear vision and pathway for their overall digitalization journey. For most companies, this is already far-reaching, starting with the introduction of digitally strengthened processes and value offerings and culminating in fully digitalized operating and business models (see Figure 19).
Digitalization usually requires fundamental reengineering and transformation of the business and typically takes place over several years. Understanding the current position on the digitalization journey helps to determine:
- What still needs to be done in terms of digitalization basics before embarking on Industrial Metaverse development (such as data availability and infrastructure)?
- What is a realistic pace of development going forward?
- What would be a feasible target destination to align with the rest of the digitalization journey?
The initial applications for Industrial Metaverse are likely to be along the “operating model” dimension — such as improving the efficiency and effectiveness of engineering, operations, maintenance, and training. Indeed, these are the measures that overlap most with Industry 4.0.
As we have shown, however, an even bigger prize that the Industrial Metaverse can deliver is to enable better and faster strategic what-if decision-making. As an example, if a company’s broader digitalization strategy encompasses, say, moving toward high-value digitalized services, changing its role in the value chain, or shifting the boundaries of its business, the use of complex system simulations will be a key enabler to help assess the impact of different business model options prior to committing investment.
Identify opportunities: Discover value-adding Industrial Metaverse opportunities & develop a roadmap
The next step involves identifying Industrial Metaverse opportunities that add value and developing a roadmap.
There are many existing applications and uses cases. In developing the roadmap, companies should consider:
- What potential applications and use cases would add greatest value? For example, in manufacturing- and process-based sectors, operations, maintenance, and supply chain business cases are often initially the most attractive.
- What is already technically feasible today versus potentially feasible in the coming years? As we have seen, although there are many currently feasible opportunities, large-scale complex industrial system simulations are still some way away.
- What is the vision and ambition for Industrial Metaverse applications in a 5+ years’ time frame? The scale of ambition may depend on the scale of digital transformation that companies may already be committed to.
- What level of detailed digital modeling is appropriate to aim for initially? Companies should usually avoid taking on very detailed modeling at the start, since this is not necessary to implement initial use cases. Detailed modeling requires large amounts of data for setup and maintenance, slowing down early progress and diminishing economic attractiveness.
- What new processes and approaches are needed to manage data? As well as the use cases themselves, the roadmap should consider what new processes are needed to access, update, analyze, interpret, and act on the required data.
Implement pilot projects: Adopt a test-and-learn approach & manage change proactively
A long-term project pipeline involving learnings capture helps to ensure that there is enough value generation to begin to self-finance the transformation process.
An agile and responsive test-and-learn approach is important in implementing the roadmap, including the following features:
- Short time to deploy. Select initial proof-of-concept projects with a relatively short payback time. Ensure that initial projects don’t require difficult and time-consuming IT platform upgrades.
- Start small and prove value. The first use cases should clearly create value that can be shown internally and externally to the company.
- Internal projects first. Start with projects that affect only limited numbers of staff and do not need involvement from external stakeholders.
- High replication potential. Try to focus on applications with high replication potential based on currently available technology. Multiple vendors should be allowed to trial their products and services.
- Manage people impacts proactively. The internal social impact of Industrial Metaverse implementation should not be underestimated. This may require employees to perform new roles and develop new skills that will require training and support.
- Be prepared to support adoption over an extended period. After deployment, employees will need support. The deployment team should not necessarily be the IT department. Using transversal teams helps to broaden involvement and embed the change. Especially for use cases involving field workers, the adoption curve is usually longer than expected. For example, AR/VR devices sometimes have a negative productivity impact initially, so strong support is key, both technically for procedures but also in terms of change management.
Build & align the ecosystem: Create a win-win situation with ecosystem partners
As we have described, the major benefits of the Industrial Metaverse are realized through involving not only internal operations but also the whole partner ecosystem and supply chain. This requires sharing much more data than is traditionally shared between commercial partners, requiring a new mindset and culture.
Moreover, developing the necessary capabilities to implement the Industrial Metaverse will require an ecosystem-based approach. This means that a key step is to actively engage with and align ecosystem partners on the project. For partners to be comfortable with sharing of internal data for this purpose, companies will have to ensure that, for example:
- The benefits for partners are assessed and demonstrated. The benefits are likely to be in areas such as faster customer response, reduced working capital, smoother customer/supplier interfaces, easier demonstration of sustainability impacts, greater overall efficiencies, and closer customer/supplier relationships.
- Data safety and security is ensured. Ecosystem partners will have to satisfy each other with regard to the safety and security of shared data.
- Standards are defined and agreed. Ecosystem partners will have to ensure that there are agreed standards and procedures for data management to manage the risks involved.
An ecosystem approach is essential for the success of the Industrial Metaverse (see Figure 20). Without it, the essential value of a digitally simulated business is hard to realize.
Appendix 1. Technology readiness levels
TRLs provide a commonly accepted means of describing technology maturity.
Appendix 2. Selected company profiles
Appendix 3. Industrial Metaverse use cases
This appendix contains more than 30 illustrative use cases for the Industrial Metaverse in its current stage of evolution (i.e., discrete digital twins). It is organized by industry and is non-exhaustive.
Notes
[1] Schuh, Günther, et al. “Using the Industrie 4.0 Maturity Index in Industry: Current Challenges, Case Studies and Trends.” Acatech, German National Academy of Science and Engineering, 2020.
[2] Taylor, Petroc. “Volume of Enterprise Data Worldwide 2020–2022, by Location.” Statista, 23 May 2022.
[3] IoT Analytics. “IoT 2022: Connected Devices Growing 18% to 14.4 Billion Globally.” IoT for All, 1 September 2022.
[4] Kallstrom, Henry. “Suppliers’ Power Is Increasing in the Automobile Industry.” Yahoo! News, 6 February 2015.
[5] The title is a reference to a Linux command line that sends a repeating value to /dev/null, which is the void — the abyss.
[6] Arthur D. Little, IoT Analytics.
[7] Arthur D. Little, Oracle, Fortune Business Insights.
[8] Arthur D. Little, Precedence Research.
[9] Arthur D. Little. “Review of Complex Systems and Their Concrete Applications in the Transportation Industry.” Presans, 2016.
[10] Arthur D. Little, GlobalNewsWire, Statista.
[11] Arthur D. Little, The Business Research Company.
[12] Arthur D. Little, Future Market Insights, Grand View Research.
[13] Arthur D. Little, The Business Research Company.
[14] For an in-depth review of quantum computing technology and impact, see: Meige, Albert, et al. “Unleashing the Business Potential of Quantum Computing.” Arthur D. Little, 2022.
[15] Miller, Rosemarie. “Microsoft’s Industrial Metaverse Aspirations Can Wait.” Forbes Digital Assets, 14 February 2023.
DOWNLOAD THE FULL REPORT
38 min read •
The Industrial Metaverse
Making the invisible visible to drive sustainable growth
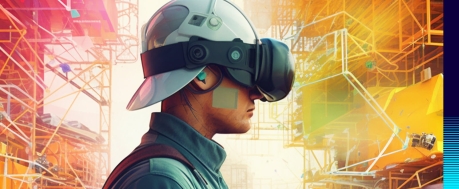
DATE
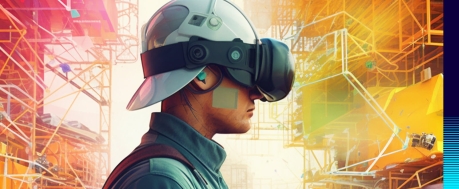
Executive Summary
“What am I? The data? The process that generates it? The relationships between the numbers?”
— Greg Egan, science fiction author, Permutation City
In business and popular media, the Metaverse hype wave is already entering its disillusionment phase, superseded by artificial intelligence (AI). Yet the Industrial Metaverse, perhaps less exciting in the popular imagination, has never really been part of the hype. Is this where the real value of the Metaverse will be realized?
There are differing views about what the Industrial Metaverse is versus the Metaverse as a whole, and how it differs from existing digital twin technologies normally considered under Industry 4.0. In this Report, we provide an evidence-based perspective, assessing current technology status, summarizing use cases and market potential, and offering recommendations for companies going forward.
We conclude that the Industrial Metaverse is best defined as a “connected whole-system digital twin with functionalities to interact with the real system in its environment, allowing decision makers to better understand the past and forecast the future.” As such, the Industrial Metaverse is a further evolution of discrete digital twin technologies that already exist today (e.g., for factories or plants) but progressively extended to ultimately represent an end-to-end, real-world industrial system, including external elements outside the company and the environment within which it operates. The Industrial Metaverse thus provides a transformative tool to elevate the use of digital simulation technology to the level of strategic decision-making. This is important for dealing with the increasing complexity and accelerated pace of development company leaders face and is especially valuable for developing effective sustainable growth strategies.
While achieving a full-scale, connected, end-to-end, whole-system digital twin may be five or more years away — especially due to development gaps in connectivity, computing capacity, and scaled-up AI — intermediate steps are possible in the short term, and many Industrial Metaverse use cases already exist. These can be grouped into four categories: (1) optimization (e.g., digital twins and augmented reality [AR] for operations/maintenance efficiency and productivity improvements); (2) training (e.g., virtual/remote training tools); (3) technical tools (e.g., design/construction/maintenance digital tools); and (4) management tools (e.g., virtual meeting/collaboration/interaction tools). The next development steps will include extending digital simulations beyond discrete physical assets toward multiple connected assets, internal processes, and functions, and finally extended upstream and downstream activities involving the entire industrial system.
We estimate the current Industrial Metaverse market to be around US $100-$150 billion, with a conservative 2030 forecast of around $400 billion but with a potential upside of more than $1 trillion. The benefits to business in terms of productivity could be multiple double-digit percentages. The growth of the Industrial Metaverse will not necessarily depend on widespread adoption of the consumer Metaverse because its utility and value for business depend more on the quality of complex system simulation and less on features such as immersivity and human-machine interface technology. Our conclusion is that the Industrial Metaverse has elements of both evolution and revolution: evolution in terms of the potential for further stepwise penetration of Industry 4.0 technologies, and revolution in terms of how the convergence of these technologies — especially connectivity, AI, complex systems simulation, and visualization powered by increasing computing capacity — has the potential to transform business productivity.
Companies need to consider their strategy for the Industrial Metaverse in the context of their broader digitalization strategy, while also considering implementation barriers. We recommend that companies consider four steps to reap the benefits:
-
Review strategy. Develop a clear picture of the digitalization strategy, journey, and current position.
-
Identify opportunities. Discover value-adding Industrial Metaverse opportunities and develop a roadmap.
-
Implement pilot projects. Adopt a test-and-learn approach and manage change proactively.
-
Build and align the ecosystem. Create a win-win situation with ecosystem partners.
Preamble
When I was a child, 10 or 11 years old, I remember thinking that if it were possible to “scan” the positions and speeds of all the atoms and molecules that make up my body at a given moment and put all this information in a computer capable of simulating all the physico-chemical reactions that govern the universe, then this digital copy would not be distinguishable from the original. There would then be two of “me” — the original, based on carbon chains, and the digital copy, whose substrate would be silicon. The copy would be as conscious as the original, and it would be just as convinced of being me.
I didn’t know it yet, but I had a materialistic approach to consciousness. I didn’t know about Heisenberg’s uncertainty principle, which prohibits knowing with infinite precision the position and the speed of the same particle. Thus, the perfect scan was therefore not possible. Not to mention the computing power required to run such a simulation is still quite far from being available. However, without knowing it, I had conceptualized what the industry would one day call “digital twins.”
Many years later, my friend David Louapre, Scientific Director at Ubisoft and creator of the popular “Science étonnante” YouTube channel, recommended that I read the science fiction book Permutation City by Australian author Greg Egan, released in 1994.
Immersing myself in Permutation City, the digital twin story of my childhood suddenly came back to me like a Proust digital madeleine. Because indeed, one of the key elements of the plot is based on the fact that in the near future, around 2050, it became possible to upload one’s consciousness to a computer. The problem is that in order for a person’s digital twin to be able to interact with a person in the real world, their simulation must run fast enough — that is, enough computing power must be available. If the computing power is insufficient, the time of the simulated person, although remaining subjectively unchanged, passes more slowly than the real time. And if, on the contrary, the computing power is excessive, the simulated world unfolds faster than the real world. It then becomes possible to foresee the future.
These are exactly the objectives that we seek to achieve with the Industrial Metaverse. The Industrial Metaverse is the extension of what has been called “Industry 4.0” for at least a decade. It is the digital twin of a complex system that allows you to project yourself through time and immerse yourself in space. It makes it possible to numerically anticipate the future consequences of a decision or an event on a complex system — whatever this system: a machine, a factory, a company, a value chain.
As we will see in this Report, the Industrial Metaverse has three major advantages over Industry 4.0:
-
Modeling and simulation of complex systems — approaches that were still part of the academic world 10 years ago and that are now changing the game in the industrial world — make it possible to create virtual what-if scenarios. The accessible data is no longer just data from the past and the present, but is now also data about the future. It becomes possible to project in time.
-
Thanks to AI and virtual reality (VR), it finally becomes possible to bring out meaning and visualize the industrial system that must be managed and thus overcome the limits of the human brain, which is not well adapted to apprehend a complex system and its emergences — the famous butterfly effect, resulting from a decision or an event.
-
Interoperability and interconnection between the physical industrial system, its digital twin, and the various stakeholders now, more and more, make it possible to manage it optimally.
Thanks to the Industrial Metaverse, it has become possible to make the invisible visible to drive sustainable growth. While ensuring economic growth, we believe that the Industrial Metaverse will be part of the solution to the climate challenge.
And, actually, it is interesting to note that an anagram of Métavers Industriel (Industrial Metaverse) is:
verdures militantes/militant greenery
This is quite intriguing and, as always, anagrams move in mysterious ways.
– Albert Meige, PhD
1
What is the context for the Industrial Metaverse?
Industrial Metaverse is a term commonly applied to the set of Metaverse applications designed for business users. In our previous Report, “The Metaverse, Beyond Fantasy,” we looked at the Metaverse as a whole, its applications, underlying technologies, and impact. In this Report, we focus specifically on Metaverse applications for businesses and enterprises, therefore excluding applications and experiences for individual consumers (e.g., gaming, entertainment, and social interaction), although there is an overlap where consumers interact with businesses at the customer interface.
Today, the Industrial Metaverse as a concept is both commonly understood and, at the same time, variously interpreted. Business managers are already well-versed in the potential of digitalization, and many are already well along the digital transformation journey. Digital models of physical products and assets, increased connectivity, and new visualizations are very much part of this journey. So what does the Industrial Metaverse really bring in addition? How significant is the creation of an immersive virtual environment to running a typical business? Is Industrial Metaverse really revolutionary, or is it in fact more evolutionary?
In this Report, we examine the background and context of the Industrial Metaverse, define what it means, set out a conceptual architecture, explore its key technological building blocks, assess its value to business both now and in the future, and propose how businesses should go about exploiting its potential. The Report is based on in-house research, client experience, and contributions from interviews with experts across industry and academia.
Industry 4.0 & the Industrial Metaverse today
The Industrial Metaverse is frequently cited as the next phase of evolution after Industry 4.0, moving from cyber-physical systems to a fully virtualized world (see Figure 1).
The term “Industry 4.0” (or the Fourth Industrial Revolution) was popularized around a decade ago and refers to the deployment of a wide range of technologies with the potential to transform industry through new cognitive tools, connectivity, virtual modeling (including digital twins), collaboration tools, and new techniques for manufacturing and supply chain, including advanced robotics and blockchain (see Figure 2).
Of these various technologies, some are especially relevant for the Industrial Metaverse. These include AI, connectivity technologies, virtualization and simulation technologies, and collaboration/interaction tools (see Chapter 3 for further exploration of key technological building blocks).
Industry 4.0 technologies already provide significant benefits to those companies that have successfully deployed them to help transform their businesses. For example, according to data from case examples in Arthur D. Little’s (ADL’s) Operational Excellence Database, these benefits are often double-digit in scale:
-
15%-30% reductions in operational capital deployed
-
10%-30% reductions in supply chain costs
-
30% increased utilization of production capacity
-
10%-40% reductions in maintenance costs
However, overall progress in achieving Industry 4.0 maturity still has a long way to go. For example, a 2020 survey of 70 German companies by Acatech that measured progress against a six-stage Industry 4.0 maturity scale showed that the vast majority of firms (80%) were still in the second stage (connectivity), with only a minority (4%) having progressed toward the next stage of creating digital twins (visibility).[1] No companies had progressed toward the last three maturity stages, which involved modeling complex interactions, simulating future-oriented what-if scenarios, or creating self-governing systems. As we will show later in this Report, these functions are key parts of what the Industrial Metaverse promises to deliver.
It is well-known that making progress on implementation of digital and Industry 4.0 technologies is challenging for any large company. This is because it typically involves fundamental transformation of the way the business operates; it is not possible to simply “bolt on” these new technologies to existing assets, business processes, and ways of working. Typical challenges include:
-
High initial investment, especially in data gathering and management
-
Limitations imposed by legacy IT systems
-
A reluctance to embrace the extent of the required business transformation
-
Difficulties in realizing the targeted business returns from digital investments within short-enough timescales
The Acatech study also highlighted common barriers toward Industry 4.0 progress, including:
-
A lack of common standards
-
Fragile information system integration
-
A reluctance to engage in interdepartmental cooperation
-
Inadequate employee involvement in change processes
If we accept that the Industrial Metaverse is a further stage of evolution beyond Industry 4.0, then it follows that its successful implementation at scale will also require overcoming these common barriers toward Industry 4.0 implementation.
Why the changing business landscape is leading to unmet needs
It is useful to consider how the business landscape has transformed since the early days of Industry 4.0. Today, as well as the constant need to further improve productivity, one of the biggest challenges facing business leaders is how to achieve sustainable net-zero impact growth. Contributing to this challenge are three key factors, as illustrated in Figure 3: complexity, acceleration, and cognition.
Complexity
Industrial systems are increasingly becoming complex systems that are subject to emergent properties making them much harder to manage. A complex system is a system having a large number of:
-
Elements (or parts)
-
Relations (connections between the parts)
-
Nested systems (systems within the system)
Examples of complex systems include cities, the climate, and living organisms. Complex systems differ from complicated systems. Complicated systems run essentially like clockwork, in a predictable manner. They may have many elements, sub-elements, and interactions, but the structure remains stable over time and they lend themselves to problem solving using structured analysis through decomposition of the elements. Up to now, most business management approaches have been based on the idea that a company’s assets, processes, and organization can be approximated to behave, at least in large part, like a complicated system.
However, increasingly this approximation is becoming unrealistic. For example, consider the recent changes in:
-
Elements. In the last two years alone, the volume of enterprise data has risen by over 40% to more than 2 petabytes.[2]
-
Relations. As a proxy for relations, the number of Internet of Things (IoT) connections grew by nearly 20% in 2022 versus 2021, reaching 14.4 billion.[3] Partner ecosystem networks have greatly increased in size and complexity in the last decade. Demonstrating this, the proportion of a typical car manufactured by third-party suppliers increased from 56% in 1985 to about 82% in 2015,[4] a proportion that is still largely the case today.
-
Nested systems. The number of nested “layers” in industrial system architectures has increased. In aerospace, for example, the number of specification elements in the latest passenger jet designs is more than 10x that of its predecessors.
What this means is that the industrial system of any large company — plants, processes, people, finance, customers, supply chain, partners, shareholders, and their environment — increasingly has to be treated as a complex system for management purposes.
Complex systems are inherently difficult to manage due to three specific properties:
-
Emergence. New, unexpected properties emerge from the interactions between the parts.
-
Non-linearity. Feedback loops between the parts may lead to exponential behaviors.
-
Resilience. A small issue within part of the system does not necessarily lead to its failure.
These properties mean that the behaviors of a complex system are very hard to predict, introducing a high degree of uncertainty into the impact of management decisions. Managers relying on simplified models of their systems to help make decisions find that those models are often inadequate. Indeed, failure to adequately recognize inherent uncertainties is one of the main reasons why new IT systems often fail to deliver the expected benefits.
Acceleration
The pace of change for business is continuing to accelerate, causing these unpredictable emergent effects to occur faster and faster. Three factors are driving this acceleration:
-
Knowledge and enabling technologies are being developed and adopted at an increasingly rapid rate, with a greater number of exponential technologies driving transformational change.
-
The lifecycle of companies and products is shortening. For example, the average lifespan of S&P 500 companies has fallen from around 35 years in the 1970s to around 20 years today. Product lifespans in many sectors are reducing, with increasing rates of disruption by new entrants and faster market penetration times.
-
Supply chains are increasingly subject to change and disruption. Ever more complex supply chains and partner ecosystems are being impacted by global and rapid disruptions such as climate change, pandemics, war in Europe, and other geopolitical instabilities. Additionally, sustainability trends such as bio-sourcing are leading to more supplier variability.
This acceleration means that companies need to be able to respond to changing circumstances more rapidly and make strategic decisions faster.
Cognition
The limitations of human cognition mean that making good decisions within these faster-moving, unpredictable systems is difficult. The human brain is not designed to deal well with complex systems — humans tend to think in a Cartesian way, breaking problems into smaller parts, which oversimplifies complexity. In these situations, humans tend to be especially susceptible to cognitive biases — relying on information that matches previous ideas and belief systems — which often leads to incorrect decisions being made.
More and more business phenomena feature nonlinear or exponential behaviors, which the human brain does not evaluate well. For example, a small error in a predicted technology development “curve fit” can cause a big discrepancy down the line, as shown in Figure 4. Examples of this include maturity in autonomous vehicles and nuclear fusion, both of which have been repeatedly overestimated, while recently AI has become exponential after many decades in the flat part of the curve.
Sustainability
Meanwhile, sustainability imperatives mean that end-to-end complex industrial system control is growing in importance. Most developed countries have set goals to be net zero between 2040–2060. This means that companies face the challenge of continuing to achieve economic growth while reducing their environmental impact to net zero.
Achieving progress on net zero impact while maintaining growth requires companies to exert control across the end-to-end complex industrial system (e.g., managing Scope 3 as well as Scope 1 and 2 emissions). This means:
-
Sharing current relevant data (e.g., operational and environmental performance data) across the entire industrial system of which they are a part, including all third parties involved.
-
Being able to predict the sustainability impacts of changes to any part of this system, including supply chain, manufacturing, distribution, sales, in-service, and end-of-life disposal/recycling.
Today this control typically is attempted by conducting discrete impact analyses and collaborating with third parties to share information and take collective action. However, achieving true end-to-end control is difficult due to a mix of technical challenges and institutional, organizational, and cultural barriers around the necessary degree of data sharing and collaboration. On the technical side, the technologies required for gathering, monitoring, analyzing, and simulating the large amounts of data necessary to predict end-to-end sustainability impacts in a complex, large-scale industrial system are not fully mature.
While none of these challenges are completely new to business, approaches used to tackle them today will increasingly be inadequate to meet the needs of the coming years, given current trends.
How the Industrial Metaverse addresses these unmet needs
Industry 4.0 technologies, including digital twins, are already providing significant benefits, but as we have discussed, without further evolution they cannot meet all of tomorrow’s needs for a variety of reasons:
-
They are mainly limited to discrete physical systems, not the “whole system.”
-
Decision-making is too static and siloed.
-
Net zero obligations on industry mean that more effective, whole-system management will be increasingly essential in the coming years.
The Industrial Metaverse provides a way to move beyond Industry 4.0 and overcome challenges around complexity, acceleration, and cognition to deliver sustainable growth by:
-
Allowing companies to make informed C-level decisions based on a dynamic, forward-looking, whole-system approach.
-
Identifying performance improvements and the impacts of change more rapidly and effectively.
-
Helping deliver on obligations to manage overall impacts and achieve sustainable growth.
In the next chapter, we will consider in more depth what Industrial Metaverse means and look at its key components.
2
What does Industrial Metaverse really mean?
In this chapter, our contention is that the concept of the Industrial Metaverse extends far beyond being merely a digital replica of a piece of machinery or a manufacturing plant. It fundamentally serves as a digital reflection of an entire corporation in its operational environment, furnishing decision-makers with insights into historical events and facilitating future predictions. The quintessential role of the Industrial Metaverse is to illuminate the unseen — make the invisible visible. It plays a crucial role in promoting a holistic perspective across compartmentalized units, enabling detailed what-if simulations that provide foresight into potential future scenarios. It unveils the intricate interrelationships within the system. Additionally, it enhances our understanding of the overall behavior and impacts of the entire system, an asset of immense importance for fostering sustainability.
Definition & framework
There are many definitions of what the term “Industrial Metaverse” means, as can be seen in the descriptions shown in Figure 5 from some of the key players contributing to its development.
While there are differing emphases, one element of broad consensus is that Industrial Metaverse is centered on the creation of digitalized models, simulations, or twins of the real world.
Another way to build a useful definition is to consider the technological building blocks that are relevant for the Industrial Metaverse, which, as we have seen, all exist already under the Industry 4.0 umbrella (see Figure 6).
As shown in Figure 6, what makes the Industrial Metaverse distinct from Industry 4.0 is the ongoing development and convergence of many of these technologies. Digital twins, with some form of real data exchange with their physical counterparts and application of AI to assist interpretation and analysis, already exist today (e.g., BMW’s iFACTORY; see also Chapter 4 and Appendix 3). It is the further development and convergence of these technologies — alongside complex system modeling and simulation, data visualization, and collaboration technologies, all enabled by greater computing power — that are leading to the Industrial Metaverse as a new concept able to meet the challenges of complexity, acceleration, and cognition as well as sustainable growth, as set out in Chapter 1.
Based on all of this, we propose the following definition for what, ultimately, the Industrial Metaverse should become:
“The Industrial Metaverse is defined as a connected whole-system digital twin with functionalities to interact with the real system in its environment, allowing decision makers to better understand the past and forecast the future.”
As defined, the Industrial Metaverse has the potential to transform how business decision makers analyze past activities and predict the future at strategic as well as operational levels. We have defined a conceptual framework for the Industrial Metaverse illustrating its key components (see Figure 7).
As shown in the figure, the core of the Industrial Metaverse is the creation and operation of a whole-system digital twin for a real-world industrial system, including all its elements, relations, and layers. Let’s consider each of the key components in turn.
Whole-system digital twin
A whole-system digital twin differs significantly from what we would call a digital twin today, which tends to focus mainly on one or more physical assets, typically plants and products. Its functionalities are representative of an end-to-end real-world industrial system, including external elements outside the company and the broader environment within which it operates. It does not simply cover a factory, plant, or product line and is persistently and bidirectionally connected to the real-world system.
It therefore needs to be constructed as a complex system to enable realistic and dynamic system behaviors to be modeled across all functions, departments, processes, assets, and players. Extensive what-if simulation functionality is a key part of the twin. Simulations are not based on past data alone, but present and future data as well.
While it may include immersive and realistic rendering of a real system, asset, and/or product, this is not the twin’s key defining feature. Visualizations may be in a variety of different forms, depending on the needs for management decision-making.
Real-world industrial system
“Real-world industrial system” refers to the set of elements, relations, and nested systems that represents a business within its environment. It comprises all the strategy, processes, organization, and resources of a business. It stretches across all business functions and assets, including external players such as supply chain and other ecosystem partners, as well as the broader external environment in which the business operates.
By its nature, a real-world industrial system is therefore a complex system, including multiple elements, relations, nested systems, and subsystems, as shown in Figure 8. It is subject to emergent and nonlinear effects.
Connect, compute, conceive & collaborate
The Industrial Metaverse needs to include four key functions to enable a whole-system digital twin that adequately represents the real-world industrial system. These are:
-
Connect — real-time bidirectional connections between the whole-system digital twin and the real-world industrial system to enable data collection and actuation back onto the system.
-
Compute — processing of very large data volumes from the real system, including analytics, complex system modeling, pattern recognition, and simulation, to enable future-scenario formulation.
-
Conceive — visualizations of both physical and nonphysical data, which may or may not be fully immersive. These visualizations interpret and present complex data in different ways, not only to simulate reality but also to facilitate understanding and to illustrate what-if scenarios.
-
Collaborate — functionality to enable a range of interactions, including between internal staff, ecosystem partners, value chain players, customers, and others. Interactions may include commercial transactions as well as everyday coworking.
How the Industrial Metaverse helps meet executive challenges
The essence of the Industrial Metaverse based on the above definition and model is to make the invisible visible. By accomplishing this, Industrial Metaverse offers some key capabilities that are very valuable to C-level decision makers (see Figure 9). Among these, it helps take a systemic view across the silos. It also helps see into the future through enabling realistic what-if simulations. And it helps to make visible the complex interactions across the system. Finally, it offers better visibility on overall whole-system behaviors and impacts, which is invaluable for achieving sustainability.
Industrial Metaverse vs. Industry 5.0
A legitimate question would be: Why speak about the Industrial Metaverse if it is just the next phase of Industry 4.0? Why not call it Industry 5.0?
The answer can be found in the fact that this next phase of Industry 4.0 shares properties with the Metaverse, as we defined it in “The Metaverse, Beyond Fantasy”:
“The future version of the Internet blending the frontiers between reality and virtuality, at the convergence of immersive spaces, collaboration platforms, social experiences, and the creator economy.”
The Industrial Metaverse shares the three key features of the Metaverse that distinguish it from the Internet — namely, immersion, interaction, and persistence — although there are also some differences of emphasis (see Figure 10).
As shown in the figure, immersion and interaction are key for the Consumer Metaverse. Immersion, in the sense of realistic rendering, is still important but not key for the Industrial and Enterprise Metaverse. For example, it is perfectly possible to interpret, analyze, and visualize complex system data without being immersed in a virtual environment. Interaction and persistence are, however, both key for the Industrial and Enterprise Metaverse — business management relies heavily on interaction, and digital twins need to be “permanent,” just like their physical counterparts.
One obvious key difference is that the Industrial Metaverse will generally comprise discrete areas only accessible to authorized persons, rather than the vision of the Metaverse, which is to create an open environment accessible to all. This lowers one of barriers toward greater Metaverse adoption, namely full interoperability.
INTERLUDE
Make the invisible visible
"My art reflects my life, a set of connected yet separate facets, moving together in the digital space.
With a PhD in computational biology from Harvard University and a master’s degree from the Ecole Nor-male Supérieure (ENS), my research centered on computational methods to understand developmental biology. In parallel, I cofounded the nonprofit organization Just One Giant Lab to promote open science across the globe.
Utilizing my expertise in data science and machine learning (ML), I developed musical and visual artistic practices that center around art-science and the question: what is data? A child of the cyberspace, I brought my fascination for immersivity into my research and my art by working with virtual reality as a medium to reveal the invisible threads, from cellular trajectories to the link between projected conscious-ness and avatar embodiment."
— Leo Blondel, Cyber Wizard
echo cos(pi) > /dev/null[5]
Objects are not always what they appear to be. When everything around us is data, what happens when we manipulate it to reveal hidden meanings? The secrets behind complexity can sometime be revealed by changing our perspective. Starting from a pyramid, applying transformation can reveal other shapes in their complexity. In the virtual realm, data is meaning, but only through the eyes of one who seeks to look beyond the visible layer.
Light is matter, matter is light
Modern science has opened the doors to digitizing information in unprecedented ways. By recording the photons emitted by molecules, matter becomes light, which then becomes bytes. Entering the cyber realm, we can shine a new light on reality. In the virtual world, the limits are bounded only by the imagination. Here, an embryo simulated from light data becomes matter again, with simulated photons coloring its invisible matter. From a transparent embryo, where light goes through only slightly scattered, the digital process allows the generation of an opaque object, upon which light will reflect: from transparency to opacity, revealing invisible shapes and processes.
3
Where is Industrial Metaverse technology today?
In this chapter, we explore the technological building blocks of the Industrial Metaverse: their maturity, challenges to overcome, and what this means for the timescales at which the full potential of the Industrial Metaverse could be realized by business.
Evolution of technologies relevant for Industrial Metaverse
It is helpful to begin by considering relevant technologies that are already in place in industry as a result of the wave of computerization and connectivity that has been ongoing for at least three decades (see Figure 11).
Technologies that involve digital simulation and virtualization, such as building information management (BIM), computer-assisted design (CAD), and digital process control, are already mature although still not fully deployed. The use of AR (e.g., for improving asset construction, maintenance, and inspection) has also become well-established, although there is still further to go in terms of adoption by industry.
In more recent years, digital twins to represent plants, factories, and products have started to be deployed, although often still more as pilot schemes and trials (see also Chapter 4 and Appendix 3 for selected use cases). These are already being used effectively to improve operational efficiency, maintenance, and quality. And in many industries, virtual simulations are already being used for operational training.
In this sense, therefore, aspects of the Industrial Metaverse already exist, overlapping with Industry 4.0, as shown in Figure 11. However, the availability of “connected whole-system digital twins with functionalities to interact with the real system in its environment,” as per our definition in Chapter 2, is still five or more years away (we provide more detailed analysis later in this chapter).
Moving toward connected whole-system digital twins
There is a fairly clear path of evolution toward whole-system digital twins that involves progressively extending and integrating digital simulation beyond discrete plant operations. Starting with technology that is already deployed today, this could mean, for example, the following steps:
-
Step 1: Digital twins for discrete, selected plant operations — including the use of AI-enabled data analytics, increased automation, AR tools for maintenance, and virtual training
-
Step 2: Digital twins created for multiple plant operations — including supply chain linkages and data integration; increasing the extent of simulation, such as by including sourcing and/or logistics optimization
-
Step 3: Digital twins created for the whole operational system — including upstream and downstream players. In this step, various management processes are increasingly integrated into the simulation in addition to purely operational processes
-
Step 4: Digital twins created for the whole end-to-end industrial system — including all management and operational processes, assets, people, and the environment
Some early examples of Step 2 already exist today. Michelin, for instance, has already piloted an approach that involves digital twin simulation for global sourcing strategy optimization, enabling the company to test and compare different strategies for optimization across a range of parameters and achieving impressive results (see sidebar “Michelin: Evolving digital twins beyond plant level to optimize global sourcing strategy”).
Technology building blocks — Maturity & further development needs
Realizing the next phase of the Industrial Metaverse at a whole-system scale will require further development and integration across a range of technology building blocks, as outlined in Figure 12, and must consider the four functions we discussed earlier:
-
Connect — requires bidirectional data exchange between the digital twin and the real-world system, comprising both “hot” current data via IoT technology and “cold” stored data via enterprise resource planning (ERP) systems.
-
Compute — requires technology to simulate complex systems and enable future scenarios to be run. It also leverages AI for data analytics and interpretation, especially for prediction.
-
Conceive — requires VR, AR, and mixed reality (MR) visualization technologies, as well as other types of visualization for complex data interpretation, sometimes integrated with AI. Advanced human-machine interface (HMI) technologies are also a key building block.
-
Collaborate — requires a range of technologies, including enterprise collaboration tools (such as Mesh and Horizon in use today), and transaction-based tools such as those often linked to Web3, including blockchain and non-fungible tokens (NFTs). API architecture that ensures easy software interoperability and data exchange is also important.
Underpinning all this functionality is the need for increased computing capability, which involves a wide range of developing technologies at different levels in the stack, from new chip design, quantum computing, and high-performance computing through to cloud and edge infrastructures and availability of low-code/no-code software solutions.
Table 1 summarizes our assessment of the maturity levels of each of these technology building blocks, including key development needs and timescales to full maturity.
In Table 1, “scale of development gap” refers to the extent of development still needed to be able to deliver the functionality envisaged for adoption of large-scale whole-system connected digital twins. “Degree of uncertainty” refers to the likelihood that current challenges may not be overcome during development. It may be seen that for the most part, the various technology building blocks have only moderate development gaps. All have only moderate degrees of uncertainty in terms of if/when current challenges will be overcome.
Overall, the picture suggests a timescale of five or more years before whole-system digital twins as described in our Industrial Metaverse definition could be realized, although in the meantime there will be continued progress with intermediate steps. AI is assessed as having a larger development gap than others mainly due to the challenges of algorithm scalability, data volumes, and computing-capacity limitations. Computing capability itself is a critical enabler for achieving very large, connected digital twin simulations. For example, many optimization problems involving multiple elements and interactions qualify as “intractable problems,” where the solution complexity rises exponentially beyond the reach of conventional computing power. Such problems would be suitable for quantum computing approaches, but this technology is probably at least 10 years away from maturity.
In the section that follows, we provide more details of the status, maturity, and implications for the Industrial Metaverse of each of these technology building blocks.
4
What is the potential value of the Industrial Metaverse to business?
In this chapter, we assess the size and future growth of the Industrial Metaverse market, provide an overview of current use cases across different functional categories and industries, and look at potential future use cases that could be possible when the Industrial Metaverse evolves further.
Market size of the Industrial Metaverse
In order to assess the size of the Industrial Metaverse market, it is important to be clear on what is included and excluded.
We have defined the Industrial Metaverse market to include the following:
-
All of the digital twin market
-
The industrial segment of the VR/AR/MR market, excluding the consumer segment
-
Most of the IoT, AI, and blockchain markets (>50%, depending on definitions)
Other Industry 4.0 technologies such as robotics and 3D printing are excluded. Computing and telecoms infrastructure markets are also excluded, as they are relevant for the entire digital market.
Based on this scope, we have looked at a range of market forecasts from different sources to indicate market potential (see Figure 13). Note that we have not conducted any bottom-up primary research.
As shown in Figure 13, the Industrial Metaverse market size in 2023 is in the $100-$150 billion range. This is smaller than many existing Industry 4.0 market estimations, which is logical given that it excludes technologies such as robotics and 3D printing.
There is much variability in forecast market growth from Industry 4.0 from different sources, and, indeed, any such forecasts should be treated with a great deal of caution. Our conservative 2030 forecast for the Industrial Metaverse market is $400 billion, although the upside could be >$1 trillion, with CAGR between 20% and 30%.
This range is wide but in line with previous ADL estimates of the broader Metaverse, which included a conservative $500 billion low end and a multi-trillion-dollar high end (see “The Metaverse, Beyond Fantasy”). Irrespective of the actual number in eight to 10 years’ time, it is clear that the market potential is very significant.
Current Industrial Metaverse use cases
Today’s Industrial Metaverse use cases can be broadly divided into four categories: optimization, training, technical tools, and management tools. Each of these has a number of subcategories, as follows:
Optimization
-
Plant/line operational-efficiency improvements
-
Predictive maintenance optimization
-
Product-quality optimization
-
Supply chain operational improvements
-
Sales operational improvement
-
Customer service improvements
Training
-
Operational staff training
-
Safety and emergency training
-
Remote training
-
Product training
Technical tools
-
Design/construction integration and design tools
-
Asset inspection/maintenance tools
-
Remote asset troubleshooting/problem-solving
-
Advanced data analytics
-
BIM
Management tools
-
Virtual meeting tools
-
Virtual collaboration/workshop tools
-
Customer-interaction tool
These use case categories stretch across most business functions of a typical company, as shown in Figure 14.
Current use cases cover the first stage of maturity of the Industrial Metaverse, overlapping with Industry 4.0. Please see Appendix 3 for a non-exhaustive list of 30 use cases based on real-world examples and organized by industry, category, and aim. These are illustrated in Figure 15.
Future Industrial Metaverse use cases
Taking an in-depth look at future use cases demonstrates that they provide major breakthrough benefits to large companies by enhancing strategic management effectiveness, as shown in Figure 16.
Although it is impossible at this stage to predict absolute numbers, it is safe to assume that they could deliver double-digit percentage performance improvements.
Current players in the Industrial Metaverse
There are hundreds of companies currently active in providing the technologies that underpin the Industrial Metaverse. Figure 17 shows a limited selection of these companies, grouped according to the technology building blocks outlined in Chapter 3. We share more detailed profiles of selected Industrial Metaverse/digital twin players in Appendix 2.
It should be noted that many players are involved in multiple technology building blocks. Obviously, many of these companies are also involved in developing the architecture of the wider Metaverse, as set out in “The Metaverse, Beyond Fantasy.”
Recent market movements in the Industrial Metaverse
The Industrial Metaverse market is still relatively immature, with predicted major growth likely to come in the medium term. Given the current pressures on the technology industry, and the Industrial Metaverse’s current lack of significant revenues, this has led to the scaling back of some initiatives as part of wider technology industry downsizing. For example:
-
In November 2022, Meta announced that it would lay off 11,000 employees, 13% of its workforce. Plans to cut a further 10,000 employees were announced in April 2023.
-
In February 2023, Microsoft shut down its 100-person Industrial Metaverse team, founded just four months earlier, as well as closing its AltspaceVR and Mixed Reality Toolkit teams. This was part of a wider 10,000-person downsizing initiative. However, a Microsoft spokesperson stated the company “remains committed to the Industrial Metaverse.”[15]
At the same time, multiple companies have recently announced partnerships:
-
Microsoft/Meta (2022) — product integration between Teams, Office, and Windows software and Meta’s VR headsets
-
Microsoft/NVIDIA (2022) — collaboration on AI, including cloud AI computing, AI applications, and services
-
Siemens/NVIDIA (2022) — connecting the NVIDIA Omniverse and Siemens Xcelerator platforms to enable full-fidelity digital twins and software-defined AI systems. The partnership is focused on the manufacturing industry
-
Unity/Hyundai (2022) — developing Meta-Factory, a Metaverse-based digital twin factory to optimize plant operation and allow virtual problem solving
From these recent events, it seems reasonable to conclude that, despite short-term setbacks and retrenchments, which reflect the prevailing global economic climate, the longer-term drivers for Industrial Metaverse growth remain strong. As we have shown, the business benefits are significant, and the barriers toward adoption are less dependent on key obstacles for the broader Metaverse, such as consumer willingness to engage in immersivity and level of realism.
5
What should companies do?
In this chapter, we draw some overall conclusions on where the Industrial Metaverse is today and look at what companies should do to ensure that they respond appropriately to ongoing developments and reap the benefits.
Overall conclusions
The extended and enhanced use of digital twins is at the core of the Industrial Metaverse. Digital twin technology has already existed for many years and is part of many digitalization and Industry 4.0 transformation programs. In this sense, therefore, the first stage of the Industrial Metaverse is already well underway. This is a source of confusion in many discussions where people question whether the Industrial Metaverse is really anything new, or if it is instead just hype. It is the extension of digital twin concepts to cover the entire organization at operational, management, and strategic levels that is the essence of what the Industrial Metaverse will bring in the coming years.
The Industrial Metaverse does not depend fully on general Metaverse adoption in order to be successful. Many Industrial Metaverse applications, such as the use of digital twins for operational improvement, are not heavily reliant on a “perfect” rendering of reality or providing a truly immersive user experience. They also do not depend on full interoperability between competing Metaverse worlds. In this sense, further progress is possible even if adoption of the consumer Metaverse fails to take off, as some observers have predicted.
The convergence of several key technologies is providing a strong driver for Industrial Metaverse development in the coming years. The convergence of further technology developments in real data connectivity, AI, data visualization, collaboration, and complex system simulation, powered by new computing capacity, acts as a powerful driver for progress in the Industrial Metaverse. This is despite shorter-term barriers resulting from global economic conditions.
The key technologies for achieving extended whole-system digital twins are not yet mature, and there are other barriers including data sharing between players. While progress is accelerating in areas such as AI, realizing the next stage of development of the Industrial Metaverse will require further development across all key technologies, as well as overcoming barriers that prevent data sharing between players in an industrial system.
Moving ahead with the Industrial Metaverse is inextricably linked to the digitalization journey. Companies therefore need to consider their strategy for the Industrial Metaverse in the context of their broader digitalization strategy. It will usually not be possible to “leapfrog” to Industrial Metaverse implementation unless digitalization is already fairly mature.
How companies should respond
Essentially, the approach companies should take for moving forward on the Industrial Metaverse should be based around normal IT or ERP system implementation good practice. Generically, we suggest considering four main steps (see Figure 18).
Review strategy: Develop a clear picture of the digitalization strategy, journey & current position
As a starting point, companies must have a clear vision and pathway for their overall digitalization journey. For most companies, this is already far-reaching, starting with the introduction of digitally strengthened processes and value offerings and culminating in fully digitalized operating and business models (see Figure 19).
Digitalization usually requires fundamental reengineering and transformation of the business and typically takes place over several years. Understanding the current position on the digitalization journey helps to determine:
- What still needs to be done in terms of digitalization basics before embarking on Industrial Metaverse development (such as data availability and infrastructure)?
- What is a realistic pace of development going forward?
- What would be a feasible target destination to align with the rest of the digitalization journey?
The initial applications for Industrial Metaverse are likely to be along the “operating model” dimension — such as improving the efficiency and effectiveness of engineering, operations, maintenance, and training. Indeed, these are the measures that overlap most with Industry 4.0.
As we have shown, however, an even bigger prize that the Industrial Metaverse can deliver is to enable better and faster strategic what-if decision-making. As an example, if a company’s broader digitalization strategy encompasses, say, moving toward high-value digitalized services, changing its role in the value chain, or shifting the boundaries of its business, the use of complex system simulations will be a key enabler to help assess the impact of different business model options prior to committing investment.
Identify opportunities: Discover value-adding Industrial Metaverse opportunities & develop a roadmap
The next step involves identifying Industrial Metaverse opportunities that add value and developing a roadmap.
There are many existing applications and uses cases. In developing the roadmap, companies should consider:
- What potential applications and use cases would add greatest value? For example, in manufacturing- and process-based sectors, operations, maintenance, and supply chain business cases are often initially the most attractive.
- What is already technically feasible today versus potentially feasible in the coming years? As we have seen, although there are many currently feasible opportunities, large-scale complex industrial system simulations are still some way away.
- What is the vision and ambition for Industrial Metaverse applications in a 5+ years’ time frame? The scale of ambition may depend on the scale of digital transformation that companies may already be committed to.
- What level of detailed digital modeling is appropriate to aim for initially? Companies should usually avoid taking on very detailed modeling at the start, since this is not necessary to implement initial use cases. Detailed modeling requires large amounts of data for setup and maintenance, slowing down early progress and diminishing economic attractiveness.
- What new processes and approaches are needed to manage data? As well as the use cases themselves, the roadmap should consider what new processes are needed to access, update, analyze, interpret, and act on the required data.
Implement pilot projects: Adopt a test-and-learn approach & manage change proactively
A long-term project pipeline involving learnings capture helps to ensure that there is enough value generation to begin to self-finance the transformation process.
An agile and responsive test-and-learn approach is important in implementing the roadmap, including the following features:
- Short time to deploy. Select initial proof-of-concept projects with a relatively short payback time. Ensure that initial projects don’t require difficult and time-consuming IT platform upgrades.
- Start small and prove value. The first use cases should clearly create value that can be shown internally and externally to the company.
- Internal projects first. Start with projects that affect only limited numbers of staff and do not need involvement from external stakeholders.
- High replication potential. Try to focus on applications with high replication potential based on currently available technology. Multiple vendors should be allowed to trial their products and services.
- Manage people impacts proactively. The internal social impact of Industrial Metaverse implementation should not be underestimated. This may require employees to perform new roles and develop new skills that will require training and support.
- Be prepared to support adoption over an extended period. After deployment, employees will need support. The deployment team should not necessarily be the IT department. Using transversal teams helps to broaden involvement and embed the change. Especially for use cases involving field workers, the adoption curve is usually longer than expected. For example, AR/VR devices sometimes have a negative productivity impact initially, so strong support is key, both technically for procedures but also in terms of change management.
Build & align the ecosystem: Create a win-win situation with ecosystem partners
As we have described, the major benefits of the Industrial Metaverse are realized through involving not only internal operations but also the whole partner ecosystem and supply chain. This requires sharing much more data than is traditionally shared between commercial partners, requiring a new mindset and culture.
Moreover, developing the necessary capabilities to implement the Industrial Metaverse will require an ecosystem-based approach. This means that a key step is to actively engage with and align ecosystem partners on the project. For partners to be comfortable with sharing of internal data for this purpose, companies will have to ensure that, for example:
- The benefits for partners are assessed and demonstrated. The benefits are likely to be in areas such as faster customer response, reduced working capital, smoother customer/supplier interfaces, easier demonstration of sustainability impacts, greater overall efficiencies, and closer customer/supplier relationships.
- Data safety and security is ensured. Ecosystem partners will have to satisfy each other with regard to the safety and security of shared data.
- Standards are defined and agreed. Ecosystem partners will have to ensure that there are agreed standards and procedures for data management to manage the risks involved.
An ecosystem approach is essential for the success of the Industrial Metaverse (see Figure 20). Without it, the essential value of a digitally simulated business is hard to realize.
Appendix 1. Technology readiness levels
TRLs provide a commonly accepted means of describing technology maturity.
Appendix 2. Selected company profiles
Appendix 3. Industrial Metaverse use cases
This appendix contains more than 30 illustrative use cases for the Industrial Metaverse in its current stage of evolution (i.e., discrete digital twins). It is organized by industry and is non-exhaustive.
Notes
[1] Schuh, Günther, et al. “Using the Industrie 4.0 Maturity Index in Industry: Current Challenges, Case Studies and Trends.” Acatech, German National Academy of Science and Engineering, 2020.
[2] Taylor, Petroc. “Volume of Enterprise Data Worldwide 2020–2022, by Location.” Statista, 23 May 2022.
[3] IoT Analytics. “IoT 2022: Connected Devices Growing 18% to 14.4 Billion Globally.” IoT for All, 1 September 2022.
[4] Kallstrom, Henry. “Suppliers’ Power Is Increasing in the Automobile Industry.” Yahoo! News, 6 February 2015.
[5] The title is a reference to a Linux command line that sends a repeating value to /dev/null, which is the void — the abyss.
[6] Arthur D. Little, IoT Analytics.
[7] Arthur D. Little, Oracle, Fortune Business Insights.
[8] Arthur D. Little, Precedence Research.
[9] Arthur D. Little. “Review of Complex Systems and Their Concrete Applications in the Transportation Industry.” Presans, 2016.
[10] Arthur D. Little, GlobalNewsWire, Statista.
[11] Arthur D. Little, The Business Research Company.
[12] Arthur D. Little, Future Market Insights, Grand View Research.
[13] Arthur D. Little, The Business Research Company.
[14] For an in-depth review of quantum computing technology and impact, see: Meige, Albert, et al. “Unleashing the Business Potential of Quantum Computing.” Arthur D. Little, 2022.
[15] Miller, Rosemarie. “Microsoft’s Industrial Metaverse Aspirations Can Wait.” Forbes Digital Assets, 14 February 2023.
DOWNLOAD THE FULL REPORT