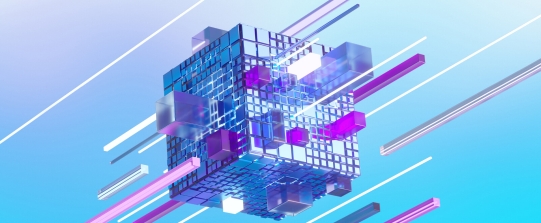
Data is at the heart of business success, enabling better decision-making, new business models, and underpinning effective artificial intelligence (AI). Sharing data across ecosystems creates more valuable data sets and delivers strategic benefits. However, external data sharing is still new to many organizations, leading to concerns about protecting data, loss of related intellectual property (IP), and ensuring regulatory compliance. Organizations can overcome these through a combination of regulatory/legal understanding and the right strategic/technology decisions.
UNDERSTANDING THE BENEFITS OF DATA SHARING
The current explosion in AI is enabling new, fast-emerging business models, based both on generative and narrow AI. In many areas, we have reached an irreversible AI singularity that has removed barriers to growth and transformation for businesses.
Data is the essential fuel for AI and is now moving beyond internal sharing to span wider ecosystems. At a basic level, external data sharing enables compliance with regulations (e.g., the EU Data Act and forthcoming EU Digital Product Passports [DPPs]). However, the opportunities and benefits of data sharing go far beyond reactive compliance, delivering benefits on an economic and societal level:
-
Enabling the creation of more powerful AI models based on a more complete picture of events, with data providing deeper insights into customers and operations.
-
Contributing to digital transformation and creating new use cases.
-
Strengthening ecosystems by sharing collective data and building transparency, trust, and coordination through a single source of truth between partners and measurement of activities, such as around sustainability targets.
-
Delivering greater organizational efficiency and creating revenue streams for all partners.
-
Allowing companies to seize opportunities around the green transition. For example, a study by Arthur D. Little (ADL) and the European Chemical Industry Council (Cefic) on the chemicals industry (see “Digital Technologies for Sustainability in the European Chemical Industry”) found that data-sharing platforms were among the four most commonly planned or currently used technologies and that big data use was expected to grow by 109% over time.
All of this leads to a beneficial flywheel effect as external data sharing matures (see Figure 1). Participants in data-sharing and collaboration initiatives can continually improve their business model and build competitive advantage, which in turn has the virtuous effect of attracting more data and partners, leading to customer and revenue growth.
MODELS TO MAKE DATA SHARING HAPPEN
Broadly, external data sharing and collaboration with partners and ecosystems can take one of three forms:
-
Data is shared as part of general business operations. Most organizations already share some data with their suppliers and customers as part of business-as-usual operations, enabling relationships to function and contractual obligations to be fulfilled. This may be expressly or implicitly covered by contracts and enables closer engagement and greater efficiency. For example, an organization might provide a subcontractor with operational information to streamline the supply of goods or services.
-
Data is pooled among participants. Organizations combine their data assets using harmonized models and standards with participants acting as both producers and consumers of data. These initiatives are normally led by a consortium, main player, or regulator. For example, Catena-X is the first collaborative, open data ecosystem for the automotive industry, linking 144 members, including BMW Group, Bosch, Siemens, Stellantis, and SAP. It aims to make it possible for data to flow from the smallest subcontractor across end-to-end value chains to automotive manufacturers. Depending on the use case, the benefits accumulate to:
-
All participants, who control the data they generate (such as within digital twins or across the supply chain).
-
The shared final customer, who controls how customer data, whether personally identifiable information (PII) or not, is used at a granular level. As an example, Schneider Electric’s EcoStruxure platform is an open and scalable Internet of Things (IoT) platform that enables interoperability across different systems and devices in the energy management and automation sectors. By leveraging data, connectivity, and analytics, EcoStruxure helps participants optimize their operations, reduce energy consumption, and drive digital transformation. On the consumer side, multiple countries, including France, Norway, and Denmark, have created health data platforms where citizens share their PII data to benefit themselves and the wider health ecosystem.
-
Wider society, with all participants pooling their data in initiatives that focus heavily on the green transition. Examples include the EU Digital Battery Passport (see “The EU data economy” below) or tracking of Scope 3 CO2 emissions across supply chains.
-
-
Unilateral data transfer. In this model, data producers transfer their data to other parties, either freely or by charging for the service. Types of this approach include:
-
Data monetization by selling data sets/insights directly or via data marketplaces. The global data monetization market is expected to reach over US $7.3 billion by 2027, with a CAGR of 24.1% from 2020–2027, according to Grandview Research. Data monetization examples include AWS Data Exchange, which has created its own data marketplace, and telecoms company SFR, which sells anonymized user location data directly through its SFR Geostatistics service.
-
Open data to drive transparency and innovation. Data is available freely under open license to citizens and businesses. This builds trust with public sector bodies and enables innovations such as open banking, data-driven smart cities, and mobility data platforms.
-
These models are driven by three key enablers:
-
Data regulations. Legislation at both an EU and national level aims to promote an open European “data economy” to ensure competitiveness and decarbonization (see sidebar “The EU data economy”). This is supported by industry-specific initiatives to enable the better use of data to solve problems at a sector/system level.
-
Business initiatives/opportunities. Emerging opportunities and regulations are accelerating the need to focus on turning data into value through external sharing.
-
Enabling technologies. Organizations can now access a full range of enabling technologies and innovative new approaches to underpin external data sharing. These include cloud technologies, data-sharing platforms, standardized data models, federated sharing, APIs, governance frameworks, and advanced cryptography solutions, which enable data owners to share data while maintaining control over it.
CHALLENGES TO DATA SHARING
Organizations often have a series of preconceived ideas around data sharing (see Figure 2).
Overcoming these requires cultural change, from the top down. Senior management needs to understand that external data sharing delivers increased value to all participants. As well as changing mindsets, organizations must tackle challenges around three main themes to drive success: strategy, governance, and technical implementation.
Strategic challenges
-
Value. Through in-depth research and analysis, organizations need to identify opportunities and understand how value can be shared with other participants to deliver value to all players.
-
Intellectual property. Organizations should put in place stringent governance processes on when data should be shared, and audit and catalog all data that may be shared to minimize the risk of disclosing confidential information/trade secrets without appropriate safeguards and limitations.
-
Competition. To guard against the possibility of handing competitive advantage to rivals, organizations should explore frameworks that balance data sharing with protection for operational/process information.
-
Regulation. Regulations shouldn’t necessarily be an obstacle to data sharing (see sidebar “Data sharing — The legal perspective”).
Governance/trust/process challenges
-
Trust. Building relationships between organizations that normally compete can appear complex. All parties within the ecosystem therefore need to work clearly and transparently to establish trust and guardrails for data sharing, while also ensuring that such arrangements do not facilitate collusion, anticompetitive foreclosure, or otherwise lead to potential competition law infringement.
-
Role. It is vital to define each player’s role, missions, and decision-making processes so that it is clear how the ecosystem operates and where value is created for each partner.
-
Ethics. While sharing and reusing external data may be perfectly legal, certain uses may be viewed as unethical. It is therefore vital to be transparent about how and why data is being shared and who has access to it.
-
Standards. On a technical level, organizations must agree on interoperability approaches, standards, and automated processes to streamline data sharing.
-
Funding. Organizations should unlock necessary investments by identifying the business benefits that external data sharing brings in terms of efficiency, innovation, new revenues, and compliance and use this to unlock financing of shared capabilities.
Technical implementation challenges
-
Platforms. At a technology level, ecosystems need to be built on well-designed, interoperable, and maintainable platforms.
-
Alignment. Ecosystem participants will have different levels of data maturity and diverse data architectures. Platforms must therefore be able to integrate with a range of technologies across different formats.
-
Innovation. To unlock future use cases, technology must be able to leverage innovative solutions, such as blockchain and multiparty computation.
-
Operationalizing customer consent. Scaling data sharing requires organizations to operationalize and scale seeking and providing customer consent. For example, Dutch energy grid operators, working with ADL, initiated a new Energy Market Facilitation Framework. This enables the permission-based sharing of personal energy usage by customers, such as when they apply for bank loans for green/solar renovations and battery storage or are provided with new services to manage electric vehicle batteries, such as predictive maintenance and optimized charging.
BEST PRACTICES IN EXTERNAL DATA SHARING
Excelling at data sharing and collaboration starts with strategic decision-making and then relies on four key capabilities to build momentum and value. Steps in the process include:
-
Start by defining the strategic objective for external data sharing and collaboration:
-
Comply with regulations.
-
Structure an ecosystem to gain competitive advantage.
-
Create new value.
-
Join an ecosystem to reinforce current position.
-
Follow other players to prevent being sidelined by future developments.
-
-
Then define the level of acceptable risk around the value of opening data versus exposing it too widely.
-
Next, identify potential data ecosystems to create, develop, or enter and define the organization’s role within it.
-
Identify potential use cases and sources of value from sharing; consider any ethical risks that need to be understood to mitigate possible reputational/revenue challenges.
-
Finally, consider on what basis the proposed sharing of data might end and what will happen to the shared data in that circumstance.
4 key capabilities
-
Understand internal data management capabilities, including cataloging current internal data landscape, competencies, and gaps.
-
Analyze external data-sharing capabilities, assessing the current partner landscape and potential opportunities.
-
Activate your ecosystem by building partnerships that augment internal capabilities, assess scope of the minimal viable consortium, establish a value proposition for each partner, and define/adopt governing roles and responsibilities.
-
Continue to monitor value creation, especially to identify new and emerging uses for data sharing.
External data sharing is at the heart of increasing competitive advantage and delivering business value, particularly around AI, compliance, and meeting sustainability targets. To achieve success, organizations must take an ecosystem approach, based on a considered understanding of strategic, regulatory, legal, and technical themes. Joining an ecosystem requires a structured approach that answers the key questions illustrated in Figure 3.
Conclusion
ENABLING TRANSFORMATION THROUGH EXTERNAL DATA SHARING
Data-driven ecosystems are reinventing the business landscape, generating new revenues, benefiting customers, enabling AI, and helping to meet sustainability targets. Organizations need to embrace external data sharing by:
-
Overcoming preconceptions, such as sharing leading to loss of control or competitive advantage.
-
Taking a strategic approach focused on their business objectives.
-
Understanding the legal and regulatory implications of sharing.
-
Identifying their own capabilities and any gaps around technology and skills.
-
Ensuring the right internal data management foundations are in place to facilitate wider sharing.
-
Understanding the best ecosystems to join to deliver strategic advantage.
-
Putting in place strong internal and external governance across the data lifecycle.
-
Identifying relevant use cases, monitoring activities and benefits, and building on successes to scale programs.